Sinc interpolation in spatial domainInterpolation vs Interpolation Filter?sinc interpolation using fftSinc interpolation looks weirdInterpolation based on sinc functiondifficulties implementing windowed sinc interpolation (C++)Absolute convergence of periodic sinc interpolationSinc interpolation of pure sine wave sampled just at Nyquist frequencyFrequency Domain Interpolation: Convolution with Sinc Function
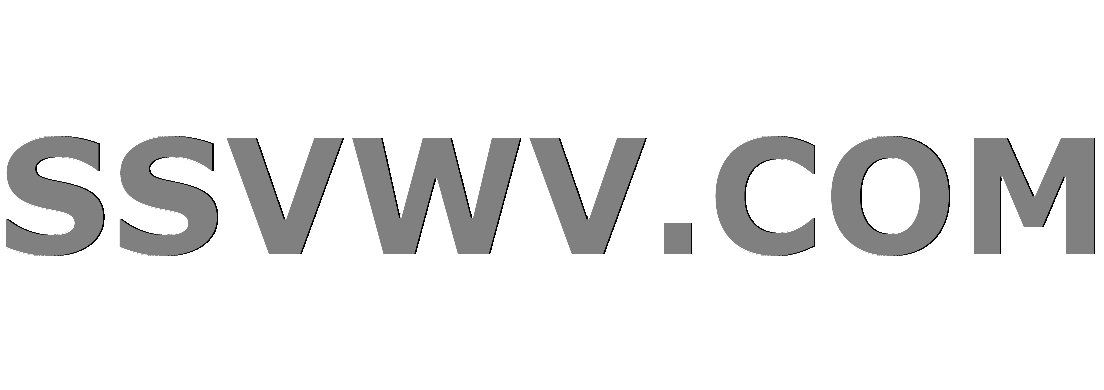
Multi tool use
Does git delete empty folders?
Is "stainless" a bulk or a surface property of stainless steel?
What's the point of writing that I know will never be used or read?
Why did St. Jerome use "virago" in Gen. 2:23?
What is the evidence on the danger of feeding whole blueberries and grapes to infants and toddlers?
!I!n!s!e!r!t! !b!e!t!w!e!e!n!
Independence of Mean and Variance of Discrete Uniform Distributions
Unsolved Problems due to Lack of Computational Power
Why don't modern jet engines use forced exhaust mixing?
Are there categories whose internal hom is somewhat 'exotic'?
Are there any OR challenges that are similar to kaggle's competitions?
Output with the same length always
Just one file echoed from an array of files
Tabularx with hline and overrightarrow vertical spacing
Quick destruction of a helium filled airship?
How can I train a replacement without them knowing?
Radix2 Fast Fourier Transform implemented in C++
What can I do to keep a threaded bolt from falling out of it’s slot
Is recepted a word?
My father gets angry everytime I pass Salam, that means I should stop saying Salam when he's around?
Why should P.I be willing to write strong LOR even if that means losing a undergraduate from his/her lab?
Playing a fast but quiet Alberti bass
Uploaded homemade mp3 to icloud music library, now "not available in my country or region"
9 hrs long transit in DEL
Sinc interpolation in spatial domain
Interpolation vs Interpolation Filter?sinc interpolation using fftSinc interpolation looks weirdInterpolation based on sinc functiondifficulties implementing windowed sinc interpolation (C++)Absolute convergence of periodic sinc interpolationSinc interpolation of pure sine wave sampled just at Nyquist frequencyFrequency Domain Interpolation: Convolution with Sinc Function
.everyoneloves__top-leaderboard:empty,.everyoneloves__mid-leaderboard:empty,.everyoneloves__bot-mid-leaderboard:empty margin-bottom:0;
$begingroup$
I have tried to perform sinc interpolation (in 1D) with the following Matlab code:
Fs=8;
T=1/Fs;
t=0:T:(1-T);
f=1;
x=sin(2*pi*f*t);
up_factor=2;
%% Deduce sinc from Fourier domain
xp=[zeros(1,5) x zeros(1,5)];
Xp=fft(xp);
door1D=abs(xp>0);
sinc1D=fftshift(abs(fft(door1D)));
%plot(door1D);hold on;plot(fftshift(abs(sinc1D)))
%% Interp with sinc in spatial domain
x_up=upsample(x,2);
%plot(x,'b*');hold on;plot(x_up,'r*');
x_up_interp=conv2(x_up,sinc1D,'same');
figure;
plot(x_up_interp./up_factor);
hold on;
plot(x);
hold on;
plot(x_up,'r+');
It seems to work (except for ripples due to the Gibbs phenomenon I guess?). However, I worked this out in a "deductive" manner and I dont completely understand the parameters (period/frequency) of the sinc. The approach was to extract the sinc from the fft of the door function. Then use that sinc as if I had computed it beforehand and convolve the upsampled (not yet interpolated) 1D signal with it.
Can someone help me understand it better? How would I built this sinc ? e.g. from the sinc() function of Matlab. And for it to work, I have convolved with abs of sinc cf. conv2(x_up,sinc1D,'same');
, but this seems strange... can someone explain/develop/correct this ?
REM.:also it is probably badly scaled, but that is another detail
fourier-transform interpolation
$endgroup$
add a comment |
$begingroup$
I have tried to perform sinc interpolation (in 1D) with the following Matlab code:
Fs=8;
T=1/Fs;
t=0:T:(1-T);
f=1;
x=sin(2*pi*f*t);
up_factor=2;
%% Deduce sinc from Fourier domain
xp=[zeros(1,5) x zeros(1,5)];
Xp=fft(xp);
door1D=abs(xp>0);
sinc1D=fftshift(abs(fft(door1D)));
%plot(door1D);hold on;plot(fftshift(abs(sinc1D)))
%% Interp with sinc in spatial domain
x_up=upsample(x,2);
%plot(x,'b*');hold on;plot(x_up,'r*');
x_up_interp=conv2(x_up,sinc1D,'same');
figure;
plot(x_up_interp./up_factor);
hold on;
plot(x);
hold on;
plot(x_up,'r+');
It seems to work (except for ripples due to the Gibbs phenomenon I guess?). However, I worked this out in a "deductive" manner and I dont completely understand the parameters (period/frequency) of the sinc. The approach was to extract the sinc from the fft of the door function. Then use that sinc as if I had computed it beforehand and convolve the upsampled (not yet interpolated) 1D signal with it.
Can someone help me understand it better? How would I built this sinc ? e.g. from the sinc() function of Matlab. And for it to work, I have convolved with abs of sinc cf. conv2(x_up,sinc1D,'same');
, but this seems strange... can someone explain/develop/correct this ?
REM.:also it is probably badly scaled, but that is another detail
fourier-transform interpolation
$endgroup$
$begingroup$
please help! :)
$endgroup$
– Machupicchu
10 hours ago
add a comment |
$begingroup$
I have tried to perform sinc interpolation (in 1D) with the following Matlab code:
Fs=8;
T=1/Fs;
t=0:T:(1-T);
f=1;
x=sin(2*pi*f*t);
up_factor=2;
%% Deduce sinc from Fourier domain
xp=[zeros(1,5) x zeros(1,5)];
Xp=fft(xp);
door1D=abs(xp>0);
sinc1D=fftshift(abs(fft(door1D)));
%plot(door1D);hold on;plot(fftshift(abs(sinc1D)))
%% Interp with sinc in spatial domain
x_up=upsample(x,2);
%plot(x,'b*');hold on;plot(x_up,'r*');
x_up_interp=conv2(x_up,sinc1D,'same');
figure;
plot(x_up_interp./up_factor);
hold on;
plot(x);
hold on;
plot(x_up,'r+');
It seems to work (except for ripples due to the Gibbs phenomenon I guess?). However, I worked this out in a "deductive" manner and I dont completely understand the parameters (period/frequency) of the sinc. The approach was to extract the sinc from the fft of the door function. Then use that sinc as if I had computed it beforehand and convolve the upsampled (not yet interpolated) 1D signal with it.
Can someone help me understand it better? How would I built this sinc ? e.g. from the sinc() function of Matlab. And for it to work, I have convolved with abs of sinc cf. conv2(x_up,sinc1D,'same');
, but this seems strange... can someone explain/develop/correct this ?
REM.:also it is probably badly scaled, but that is another detail
fourier-transform interpolation
$endgroup$
I have tried to perform sinc interpolation (in 1D) with the following Matlab code:
Fs=8;
T=1/Fs;
t=0:T:(1-T);
f=1;
x=sin(2*pi*f*t);
up_factor=2;
%% Deduce sinc from Fourier domain
xp=[zeros(1,5) x zeros(1,5)];
Xp=fft(xp);
door1D=abs(xp>0);
sinc1D=fftshift(abs(fft(door1D)));
%plot(door1D);hold on;plot(fftshift(abs(sinc1D)))
%% Interp with sinc in spatial domain
x_up=upsample(x,2);
%plot(x,'b*');hold on;plot(x_up,'r*');
x_up_interp=conv2(x_up,sinc1D,'same');
figure;
plot(x_up_interp./up_factor);
hold on;
plot(x);
hold on;
plot(x_up,'r+');
It seems to work (except for ripples due to the Gibbs phenomenon I guess?). However, I worked this out in a "deductive" manner and I dont completely understand the parameters (period/frequency) of the sinc. The approach was to extract the sinc from the fft of the door function. Then use that sinc as if I had computed it beforehand and convolve the upsampled (not yet interpolated) 1D signal with it.
Can someone help me understand it better? How would I built this sinc ? e.g. from the sinc() function of Matlab. And for it to work, I have convolved with abs of sinc cf. conv2(x_up,sinc1D,'same');
, but this seems strange... can someone explain/develop/correct this ?
REM.:also it is probably badly scaled, but that is another detail
fourier-transform interpolation
fourier-transform interpolation
asked 11 hours ago
MachupicchuMachupicchu
1121 silver badge11 bronze badges
1121 silver badge11 bronze badges
$begingroup$
please help! :)
$endgroup$
– Machupicchu
10 hours ago
add a comment |
$begingroup$
please help! :)
$endgroup$
– Machupicchu
10 hours ago
$begingroup$
please help! :)
$endgroup$
– Machupicchu
10 hours ago
$begingroup$
please help! :)
$endgroup$
– Machupicchu
10 hours ago
add a comment |
2 Answers
2
active
oldest
votes
$begingroup$
The sinc function actually represents an ideal (brickwall) lowpass filter that's used to complete the interpolation process after the data has been expanded (zero stuffed) properly. So let me outline the time domain approach here:
Assume you have data samples $x[n]$ of length $N$, and you want to upsample this by the integer factor of $L$, yielding a new interpolated data $y[n]$ of length $M = L times N $ samples.
The first stage is to zero stuff the input $x[n]$; i.e., expand it by $L$ by the expander block :
$$ x[n] longrightarrow boxed uparrow L longrightarrow x_e[n] $$
where $x_e[n]$ is related to $x[n]$ by the following:
$$ x_e[n] = begincases x[n] ~~~,~~~ n = 0, pm L, pm 2L... \ ~~~ 0 ~~ ~~~,~~~textotherwise endcases $$
Then, to complete the interpolation process and fill in the empty (zeroed) samples of $x_e[n]$, one has to lowpass filter $x_e[n]$ by an ideal lowpass filter $h[n]$ with the following frequency domain definition $H(omega)$ :
$$ H(omega) = begincases < fracpiL \ ~ 0 ~ ~~~,~~~textotherwise endcases $$
The impulse response $h[n]$ of this ideal filter is computed by the inverse discrete-time Fourier transform of $H(omega)$ and is given by
$$ h[n] = L frac sin( fracpiL n) pi n $$
This is an infitely long and non-causal filter, and thus cannot be implemented in this form. (See Hilmar's comments) Practically it's truncated and weighted by a window function, for example by a Hamming or Kaiser window.
The following MATLAB / OCTAVE code represents designing the filter and applying it into data in time domain:
L = 5; % interpolation factor
N = 500; % data length
x = hamming(N)'.*randn(1,N); % generate bandlimited data...
% expanded signal
xe = zeros(1,N*L);
xe(1:L:end) = x; % generate th expanded signal
% interpolation sinc filter
n = -32:32; % timing index
h = L*sin(pi*n/L)./(pi*n); % ideal lowpass filter
h(33) = L; % fill in the zero divison sample
h = hamming(65)'.*h; % apply weighting window on h[n]
% interpolate:
y = filter(h,1,xe); % y[n] is the interpolated signal
$endgroup$
$begingroup$
great answer, thanks. This is exactly what I was looking for, and I think it will be useful to many.
$endgroup$
– Machupicchu
6 hours ago
$begingroup$
So the lowpass filter is needed because the "useful" spectrum of xis shrinked when comparing x and xe (from an img proc lecture), and aliases apprear around it. (not sure I express myself correctly?). What is exactly this shinking factor ?
$endgroup$
– Machupicchu
6 hours ago
$begingroup$
also, why did you choose -32:32 for sinc? Is is arbitrary?
$endgroup$
– Machupicchu
6 hours ago
$begingroup$
last question, also would it be possible for you to say intuitively why the sinc interpolation is the ideal one? ( I remember as you just mentioned that the ideal lowpass filter is a brickwall to cut all freq outside it, in Fourier domain, and therefore a convolution with sinc in time/spatial domain, but "intuitively" compared to nearest neighbor interp for example why is is so much better?
$endgroup$
– Machupicchu
5 hours ago
$begingroup$
yes, yes&no and yes. 1- As a technical terminology, the spectrum of $x_e[n]$ contains images rather than aliases and the lowpass filter removes all the images outside the frequency band $|omega| < pi/L$; the shrinking factor is $L$. 2-Length of the filter is arbitrary but not so, to get a better approximation it must be long enough. 3- Sinc() interpolator is the ideal one because it corresponds to the impulse response of the perfect interpolation filter and derived based on inverse Fourier transform it. Nearest neighbor is not a perfect interpolator by definition.
$endgroup$
– Fat32
4 hours ago
|
show 2 more comments
$begingroup$
Sinc() interpolation looks nice on paper or in text books but in practice it's rarely a good solution. The main problem is that the sinc() impulse response is infinitely long and it's not causal. Not only does it have infinite length, but it also decays only very slowly with time, so you typically need a large number of samples to get a decent accuracy.
This, in turn, results in long latency, high computational cost and fairly large "transition" areas at the beginning and end of the output.
$endgroup$
$begingroup$
i think, in practice, a Kaiser-windowed sinc() interpolation can be very good. nearly always, not rarely. nearly as good as Parks-McClellan (firpm()
) or Least Squares (firls()
) and sometimes better, if you compare apples to apples. also sometimesfirpm()
chokes for kernels bigger than 2048 andfirls()
chokes for kernels bigger than 4096. but the kernal size is the product of the number of phases (sometimes i like that as high as 512) and the number of FIR taps (like 32 or 64). but you can make it as big as you want withkaiser()
andsinc()
.
$endgroup$
– robert bristow-johnson
6 hours ago
$begingroup$
so the choice by @Fat32 range of -32 to 32 is arbitrary ?
$endgroup$
– Machupicchu
6 hours ago
$begingroup$
not arbitrary, it's just that we know from FIR filter design that we will need about 32 taps (which means you're looking at 16 samples on the left and 16 samples on the right) to do a decent job of high-quality interpolation of audio. but to do a really good job of it, you might need 64 taps. the number of FIR taps times the number of phases in the polyphase FIR filter is the total length of the the FIR that you would need to design using tools likefirpm()
orfirls()
in MATLAB.
$endgroup$
– robert bristow-johnson
2 hours ago
add a comment |
Your Answer
StackExchange.ready(function()
var channelOptions =
tags: "".split(" "),
id: "295"
;
initTagRenderer("".split(" "), "".split(" "), channelOptions);
StackExchange.using("externalEditor", function()
// Have to fire editor after snippets, if snippets enabled
if (StackExchange.settings.snippets.snippetsEnabled)
StackExchange.using("snippets", function()
createEditor();
);
else
createEditor();
);
function createEditor()
StackExchange.prepareEditor(
heartbeatType: 'answer',
autoActivateHeartbeat: false,
convertImagesToLinks: false,
noModals: true,
showLowRepImageUploadWarning: true,
reputationToPostImages: null,
bindNavPrevention: true,
postfix: "",
imageUploader:
brandingHtml: "Powered by u003ca class="icon-imgur-white" href="https://imgur.com/"u003eu003c/au003e",
contentPolicyHtml: "User contributions licensed under u003ca href="https://creativecommons.org/licenses/by-sa/3.0/"u003ecc by-sa 3.0 with attribution requiredu003c/au003e u003ca href="https://stackoverflow.com/legal/content-policy"u003e(content policy)u003c/au003e",
allowUrls: true
,
noCode: true, onDemand: true,
discardSelector: ".discard-answer"
,immediatelyShowMarkdownHelp:true
);
);
Sign up or log in
StackExchange.ready(function ()
StackExchange.helpers.onClickDraftSave('#login-link');
);
Sign up using Google
Sign up using Facebook
Sign up using Email and Password
Post as a guest
Required, but never shown
StackExchange.ready(
function ()
StackExchange.openid.initPostLogin('.new-post-login', 'https%3a%2f%2fdsp.stackexchange.com%2fquestions%2f60179%2fsinc-interpolation-in-spatial-domain%23new-answer', 'question_page');
);
Post as a guest
Required, but never shown
2 Answers
2
active
oldest
votes
2 Answers
2
active
oldest
votes
active
oldest
votes
active
oldest
votes
$begingroup$
The sinc function actually represents an ideal (brickwall) lowpass filter that's used to complete the interpolation process after the data has been expanded (zero stuffed) properly. So let me outline the time domain approach here:
Assume you have data samples $x[n]$ of length $N$, and you want to upsample this by the integer factor of $L$, yielding a new interpolated data $y[n]$ of length $M = L times N $ samples.
The first stage is to zero stuff the input $x[n]$; i.e., expand it by $L$ by the expander block :
$$ x[n] longrightarrow boxed uparrow L longrightarrow x_e[n] $$
where $x_e[n]$ is related to $x[n]$ by the following:
$$ x_e[n] = begincases x[n] ~~~,~~~ n = 0, pm L, pm 2L... \ ~~~ 0 ~~ ~~~,~~~textotherwise endcases $$
Then, to complete the interpolation process and fill in the empty (zeroed) samples of $x_e[n]$, one has to lowpass filter $x_e[n]$ by an ideal lowpass filter $h[n]$ with the following frequency domain definition $H(omega)$ :
$$ H(omega) = begincases < fracpiL \ ~ 0 ~ ~~~,~~~textotherwise endcases $$
The impulse response $h[n]$ of this ideal filter is computed by the inverse discrete-time Fourier transform of $H(omega)$ and is given by
$$ h[n] = L frac sin( fracpiL n) pi n $$
This is an infitely long and non-causal filter, and thus cannot be implemented in this form. (See Hilmar's comments) Practically it's truncated and weighted by a window function, for example by a Hamming or Kaiser window.
The following MATLAB / OCTAVE code represents designing the filter and applying it into data in time domain:
L = 5; % interpolation factor
N = 500; % data length
x = hamming(N)'.*randn(1,N); % generate bandlimited data...
% expanded signal
xe = zeros(1,N*L);
xe(1:L:end) = x; % generate th expanded signal
% interpolation sinc filter
n = -32:32; % timing index
h = L*sin(pi*n/L)./(pi*n); % ideal lowpass filter
h(33) = L; % fill in the zero divison sample
h = hamming(65)'.*h; % apply weighting window on h[n]
% interpolate:
y = filter(h,1,xe); % y[n] is the interpolated signal
$endgroup$
$begingroup$
great answer, thanks. This is exactly what I was looking for, and I think it will be useful to many.
$endgroup$
– Machupicchu
6 hours ago
$begingroup$
So the lowpass filter is needed because the "useful" spectrum of xis shrinked when comparing x and xe (from an img proc lecture), and aliases apprear around it. (not sure I express myself correctly?). What is exactly this shinking factor ?
$endgroup$
– Machupicchu
6 hours ago
$begingroup$
also, why did you choose -32:32 for sinc? Is is arbitrary?
$endgroup$
– Machupicchu
6 hours ago
$begingroup$
last question, also would it be possible for you to say intuitively why the sinc interpolation is the ideal one? ( I remember as you just mentioned that the ideal lowpass filter is a brickwall to cut all freq outside it, in Fourier domain, and therefore a convolution with sinc in time/spatial domain, but "intuitively" compared to nearest neighbor interp for example why is is so much better?
$endgroup$
– Machupicchu
5 hours ago
$begingroup$
yes, yes&no and yes. 1- As a technical terminology, the spectrum of $x_e[n]$ contains images rather than aliases and the lowpass filter removes all the images outside the frequency band $|omega| < pi/L$; the shrinking factor is $L$. 2-Length of the filter is arbitrary but not so, to get a better approximation it must be long enough. 3- Sinc() interpolator is the ideal one because it corresponds to the impulse response of the perfect interpolation filter and derived based on inverse Fourier transform it. Nearest neighbor is not a perfect interpolator by definition.
$endgroup$
– Fat32
4 hours ago
|
show 2 more comments
$begingroup$
The sinc function actually represents an ideal (brickwall) lowpass filter that's used to complete the interpolation process after the data has been expanded (zero stuffed) properly. So let me outline the time domain approach here:
Assume you have data samples $x[n]$ of length $N$, and you want to upsample this by the integer factor of $L$, yielding a new interpolated data $y[n]$ of length $M = L times N $ samples.
The first stage is to zero stuff the input $x[n]$; i.e., expand it by $L$ by the expander block :
$$ x[n] longrightarrow boxed uparrow L longrightarrow x_e[n] $$
where $x_e[n]$ is related to $x[n]$ by the following:
$$ x_e[n] = begincases x[n] ~~~,~~~ n = 0, pm L, pm 2L... \ ~~~ 0 ~~ ~~~,~~~textotherwise endcases $$
Then, to complete the interpolation process and fill in the empty (zeroed) samples of $x_e[n]$, one has to lowpass filter $x_e[n]$ by an ideal lowpass filter $h[n]$ with the following frequency domain definition $H(omega)$ :
$$ H(omega) = begincases < fracpiL \ ~ 0 ~ ~~~,~~~textotherwise endcases $$
The impulse response $h[n]$ of this ideal filter is computed by the inverse discrete-time Fourier transform of $H(omega)$ and is given by
$$ h[n] = L frac sin( fracpiL n) pi n $$
This is an infitely long and non-causal filter, and thus cannot be implemented in this form. (See Hilmar's comments) Practically it's truncated and weighted by a window function, for example by a Hamming or Kaiser window.
The following MATLAB / OCTAVE code represents designing the filter and applying it into data in time domain:
L = 5; % interpolation factor
N = 500; % data length
x = hamming(N)'.*randn(1,N); % generate bandlimited data...
% expanded signal
xe = zeros(1,N*L);
xe(1:L:end) = x; % generate th expanded signal
% interpolation sinc filter
n = -32:32; % timing index
h = L*sin(pi*n/L)./(pi*n); % ideal lowpass filter
h(33) = L; % fill in the zero divison sample
h = hamming(65)'.*h; % apply weighting window on h[n]
% interpolate:
y = filter(h,1,xe); % y[n] is the interpolated signal
$endgroup$
$begingroup$
great answer, thanks. This is exactly what I was looking for, and I think it will be useful to many.
$endgroup$
– Machupicchu
6 hours ago
$begingroup$
So the lowpass filter is needed because the "useful" spectrum of xis shrinked when comparing x and xe (from an img proc lecture), and aliases apprear around it. (not sure I express myself correctly?). What is exactly this shinking factor ?
$endgroup$
– Machupicchu
6 hours ago
$begingroup$
also, why did you choose -32:32 for sinc? Is is arbitrary?
$endgroup$
– Machupicchu
6 hours ago
$begingroup$
last question, also would it be possible for you to say intuitively why the sinc interpolation is the ideal one? ( I remember as you just mentioned that the ideal lowpass filter is a brickwall to cut all freq outside it, in Fourier domain, and therefore a convolution with sinc in time/spatial domain, but "intuitively" compared to nearest neighbor interp for example why is is so much better?
$endgroup$
– Machupicchu
5 hours ago
$begingroup$
yes, yes&no and yes. 1- As a technical terminology, the spectrum of $x_e[n]$ contains images rather than aliases and the lowpass filter removes all the images outside the frequency band $|omega| < pi/L$; the shrinking factor is $L$. 2-Length of the filter is arbitrary but not so, to get a better approximation it must be long enough. 3- Sinc() interpolator is the ideal one because it corresponds to the impulse response of the perfect interpolation filter and derived based on inverse Fourier transform it. Nearest neighbor is not a perfect interpolator by definition.
$endgroup$
– Fat32
4 hours ago
|
show 2 more comments
$begingroup$
The sinc function actually represents an ideal (brickwall) lowpass filter that's used to complete the interpolation process after the data has been expanded (zero stuffed) properly. So let me outline the time domain approach here:
Assume you have data samples $x[n]$ of length $N$, and you want to upsample this by the integer factor of $L$, yielding a new interpolated data $y[n]$ of length $M = L times N $ samples.
The first stage is to zero stuff the input $x[n]$; i.e., expand it by $L$ by the expander block :
$$ x[n] longrightarrow boxed uparrow L longrightarrow x_e[n] $$
where $x_e[n]$ is related to $x[n]$ by the following:
$$ x_e[n] = begincases x[n] ~~~,~~~ n = 0, pm L, pm 2L... \ ~~~ 0 ~~ ~~~,~~~textotherwise endcases $$
Then, to complete the interpolation process and fill in the empty (zeroed) samples of $x_e[n]$, one has to lowpass filter $x_e[n]$ by an ideal lowpass filter $h[n]$ with the following frequency domain definition $H(omega)$ :
$$ H(omega) = begincases < fracpiL \ ~ 0 ~ ~~~,~~~textotherwise endcases $$
The impulse response $h[n]$ of this ideal filter is computed by the inverse discrete-time Fourier transform of $H(omega)$ and is given by
$$ h[n] = L frac sin( fracpiL n) pi n $$
This is an infitely long and non-causal filter, and thus cannot be implemented in this form. (See Hilmar's comments) Practically it's truncated and weighted by a window function, for example by a Hamming or Kaiser window.
The following MATLAB / OCTAVE code represents designing the filter and applying it into data in time domain:
L = 5; % interpolation factor
N = 500; % data length
x = hamming(N)'.*randn(1,N); % generate bandlimited data...
% expanded signal
xe = zeros(1,N*L);
xe(1:L:end) = x; % generate th expanded signal
% interpolation sinc filter
n = -32:32; % timing index
h = L*sin(pi*n/L)./(pi*n); % ideal lowpass filter
h(33) = L; % fill in the zero divison sample
h = hamming(65)'.*h; % apply weighting window on h[n]
% interpolate:
y = filter(h,1,xe); % y[n] is the interpolated signal
$endgroup$
The sinc function actually represents an ideal (brickwall) lowpass filter that's used to complete the interpolation process after the data has been expanded (zero stuffed) properly. So let me outline the time domain approach here:
Assume you have data samples $x[n]$ of length $N$, and you want to upsample this by the integer factor of $L$, yielding a new interpolated data $y[n]$ of length $M = L times N $ samples.
The first stage is to zero stuff the input $x[n]$; i.e., expand it by $L$ by the expander block :
$$ x[n] longrightarrow boxed uparrow L longrightarrow x_e[n] $$
where $x_e[n]$ is related to $x[n]$ by the following:
$$ x_e[n] = begincases x[n] ~~~,~~~ n = 0, pm L, pm 2L... \ ~~~ 0 ~~ ~~~,~~~textotherwise endcases $$
Then, to complete the interpolation process and fill in the empty (zeroed) samples of $x_e[n]$, one has to lowpass filter $x_e[n]$ by an ideal lowpass filter $h[n]$ with the following frequency domain definition $H(omega)$ :
$$ H(omega) = begincases < fracpiL \ ~ 0 ~ ~~~,~~~textotherwise endcases $$
The impulse response $h[n]$ of this ideal filter is computed by the inverse discrete-time Fourier transform of $H(omega)$ and is given by
$$ h[n] = L frac sin( fracpiL n) pi n $$
This is an infitely long and non-causal filter, and thus cannot be implemented in this form. (See Hilmar's comments) Practically it's truncated and weighted by a window function, for example by a Hamming or Kaiser window.
The following MATLAB / OCTAVE code represents designing the filter and applying it into data in time domain:
L = 5; % interpolation factor
N = 500; % data length
x = hamming(N)'.*randn(1,N); % generate bandlimited data...
% expanded signal
xe = zeros(1,N*L);
xe(1:L:end) = x; % generate th expanded signal
% interpolation sinc filter
n = -32:32; % timing index
h = L*sin(pi*n/L)./(pi*n); % ideal lowpass filter
h(33) = L; % fill in the zero divison sample
h = hamming(65)'.*h; % apply weighting window on h[n]
% interpolate:
y = filter(h,1,xe); % y[n] is the interpolated signal
answered 7 hours ago


Fat32Fat32
17.7k3 gold badges14 silver badges34 bronze badges
17.7k3 gold badges14 silver badges34 bronze badges
$begingroup$
great answer, thanks. This is exactly what I was looking for, and I think it will be useful to many.
$endgroup$
– Machupicchu
6 hours ago
$begingroup$
So the lowpass filter is needed because the "useful" spectrum of xis shrinked when comparing x and xe (from an img proc lecture), and aliases apprear around it. (not sure I express myself correctly?). What is exactly this shinking factor ?
$endgroup$
– Machupicchu
6 hours ago
$begingroup$
also, why did you choose -32:32 for sinc? Is is arbitrary?
$endgroup$
– Machupicchu
6 hours ago
$begingroup$
last question, also would it be possible for you to say intuitively why the sinc interpolation is the ideal one? ( I remember as you just mentioned that the ideal lowpass filter is a brickwall to cut all freq outside it, in Fourier domain, and therefore a convolution with sinc in time/spatial domain, but "intuitively" compared to nearest neighbor interp for example why is is so much better?
$endgroup$
– Machupicchu
5 hours ago
$begingroup$
yes, yes&no and yes. 1- As a technical terminology, the spectrum of $x_e[n]$ contains images rather than aliases and the lowpass filter removes all the images outside the frequency band $|omega| < pi/L$; the shrinking factor is $L$. 2-Length of the filter is arbitrary but not so, to get a better approximation it must be long enough. 3- Sinc() interpolator is the ideal one because it corresponds to the impulse response of the perfect interpolation filter and derived based on inverse Fourier transform it. Nearest neighbor is not a perfect interpolator by definition.
$endgroup$
– Fat32
4 hours ago
|
show 2 more comments
$begingroup$
great answer, thanks. This is exactly what I was looking for, and I think it will be useful to many.
$endgroup$
– Machupicchu
6 hours ago
$begingroup$
So the lowpass filter is needed because the "useful" spectrum of xis shrinked when comparing x and xe (from an img proc lecture), and aliases apprear around it. (not sure I express myself correctly?). What is exactly this shinking factor ?
$endgroup$
– Machupicchu
6 hours ago
$begingroup$
also, why did you choose -32:32 for sinc? Is is arbitrary?
$endgroup$
– Machupicchu
6 hours ago
$begingroup$
last question, also would it be possible for you to say intuitively why the sinc interpolation is the ideal one? ( I remember as you just mentioned that the ideal lowpass filter is a brickwall to cut all freq outside it, in Fourier domain, and therefore a convolution with sinc in time/spatial domain, but "intuitively" compared to nearest neighbor interp for example why is is so much better?
$endgroup$
– Machupicchu
5 hours ago
$begingroup$
yes, yes&no and yes. 1- As a technical terminology, the spectrum of $x_e[n]$ contains images rather than aliases and the lowpass filter removes all the images outside the frequency band $|omega| < pi/L$; the shrinking factor is $L$. 2-Length of the filter is arbitrary but not so, to get a better approximation it must be long enough. 3- Sinc() interpolator is the ideal one because it corresponds to the impulse response of the perfect interpolation filter and derived based on inverse Fourier transform it. Nearest neighbor is not a perfect interpolator by definition.
$endgroup$
– Fat32
4 hours ago
$begingroup$
great answer, thanks. This is exactly what I was looking for, and I think it will be useful to many.
$endgroup$
– Machupicchu
6 hours ago
$begingroup$
great answer, thanks. This is exactly what I was looking for, and I think it will be useful to many.
$endgroup$
– Machupicchu
6 hours ago
$begingroup$
So the lowpass filter is needed because the "useful" spectrum of xis shrinked when comparing x and xe (from an img proc lecture), and aliases apprear around it. (not sure I express myself correctly?). What is exactly this shinking factor ?
$endgroup$
– Machupicchu
6 hours ago
$begingroup$
So the lowpass filter is needed because the "useful" spectrum of xis shrinked when comparing x and xe (from an img proc lecture), and aliases apprear around it. (not sure I express myself correctly?). What is exactly this shinking factor ?
$endgroup$
– Machupicchu
6 hours ago
$begingroup$
also, why did you choose -32:32 for sinc? Is is arbitrary?
$endgroup$
– Machupicchu
6 hours ago
$begingroup$
also, why did you choose -32:32 for sinc? Is is arbitrary?
$endgroup$
– Machupicchu
6 hours ago
$begingroup$
last question, also would it be possible for you to say intuitively why the sinc interpolation is the ideal one? ( I remember as you just mentioned that the ideal lowpass filter is a brickwall to cut all freq outside it, in Fourier domain, and therefore a convolution with sinc in time/spatial domain, but "intuitively" compared to nearest neighbor interp for example why is is so much better?
$endgroup$
– Machupicchu
5 hours ago
$begingroup$
last question, also would it be possible for you to say intuitively why the sinc interpolation is the ideal one? ( I remember as you just mentioned that the ideal lowpass filter is a brickwall to cut all freq outside it, in Fourier domain, and therefore a convolution with sinc in time/spatial domain, but "intuitively" compared to nearest neighbor interp for example why is is so much better?
$endgroup$
– Machupicchu
5 hours ago
$begingroup$
yes, yes&no and yes. 1- As a technical terminology, the spectrum of $x_e[n]$ contains images rather than aliases and the lowpass filter removes all the images outside the frequency band $|omega| < pi/L$; the shrinking factor is $L$. 2-Length of the filter is arbitrary but not so, to get a better approximation it must be long enough. 3- Sinc() interpolator is the ideal one because it corresponds to the impulse response of the perfect interpolation filter and derived based on inverse Fourier transform it. Nearest neighbor is not a perfect interpolator by definition.
$endgroup$
– Fat32
4 hours ago
$begingroup$
yes, yes&no and yes. 1- As a technical terminology, the spectrum of $x_e[n]$ contains images rather than aliases and the lowpass filter removes all the images outside the frequency band $|omega| < pi/L$; the shrinking factor is $L$. 2-Length of the filter is arbitrary but not so, to get a better approximation it must be long enough. 3- Sinc() interpolator is the ideal one because it corresponds to the impulse response of the perfect interpolation filter and derived based on inverse Fourier transform it. Nearest neighbor is not a perfect interpolator by definition.
$endgroup$
– Fat32
4 hours ago
|
show 2 more comments
$begingroup$
Sinc() interpolation looks nice on paper or in text books but in practice it's rarely a good solution. The main problem is that the sinc() impulse response is infinitely long and it's not causal. Not only does it have infinite length, but it also decays only very slowly with time, so you typically need a large number of samples to get a decent accuracy.
This, in turn, results in long latency, high computational cost and fairly large "transition" areas at the beginning and end of the output.
$endgroup$
$begingroup$
i think, in practice, a Kaiser-windowed sinc() interpolation can be very good. nearly always, not rarely. nearly as good as Parks-McClellan (firpm()
) or Least Squares (firls()
) and sometimes better, if you compare apples to apples. also sometimesfirpm()
chokes for kernels bigger than 2048 andfirls()
chokes for kernels bigger than 4096. but the kernal size is the product of the number of phases (sometimes i like that as high as 512) and the number of FIR taps (like 32 or 64). but you can make it as big as you want withkaiser()
andsinc()
.
$endgroup$
– robert bristow-johnson
6 hours ago
$begingroup$
so the choice by @Fat32 range of -32 to 32 is arbitrary ?
$endgroup$
– Machupicchu
6 hours ago
$begingroup$
not arbitrary, it's just that we know from FIR filter design that we will need about 32 taps (which means you're looking at 16 samples on the left and 16 samples on the right) to do a decent job of high-quality interpolation of audio. but to do a really good job of it, you might need 64 taps. the number of FIR taps times the number of phases in the polyphase FIR filter is the total length of the the FIR that you would need to design using tools likefirpm()
orfirls()
in MATLAB.
$endgroup$
– robert bristow-johnson
2 hours ago
add a comment |
$begingroup$
Sinc() interpolation looks nice on paper or in text books but in practice it's rarely a good solution. The main problem is that the sinc() impulse response is infinitely long and it's not causal. Not only does it have infinite length, but it also decays only very slowly with time, so you typically need a large number of samples to get a decent accuracy.
This, in turn, results in long latency, high computational cost and fairly large "transition" areas at the beginning and end of the output.
$endgroup$
$begingroup$
i think, in practice, a Kaiser-windowed sinc() interpolation can be very good. nearly always, not rarely. nearly as good as Parks-McClellan (firpm()
) or Least Squares (firls()
) and sometimes better, if you compare apples to apples. also sometimesfirpm()
chokes for kernels bigger than 2048 andfirls()
chokes for kernels bigger than 4096. but the kernal size is the product of the number of phases (sometimes i like that as high as 512) and the number of FIR taps (like 32 or 64). but you can make it as big as you want withkaiser()
andsinc()
.
$endgroup$
– robert bristow-johnson
6 hours ago
$begingroup$
so the choice by @Fat32 range of -32 to 32 is arbitrary ?
$endgroup$
– Machupicchu
6 hours ago
$begingroup$
not arbitrary, it's just that we know from FIR filter design that we will need about 32 taps (which means you're looking at 16 samples on the left and 16 samples on the right) to do a decent job of high-quality interpolation of audio. but to do a really good job of it, you might need 64 taps. the number of FIR taps times the number of phases in the polyphase FIR filter is the total length of the the FIR that you would need to design using tools likefirpm()
orfirls()
in MATLAB.
$endgroup$
– robert bristow-johnson
2 hours ago
add a comment |
$begingroup$
Sinc() interpolation looks nice on paper or in text books but in practice it's rarely a good solution. The main problem is that the sinc() impulse response is infinitely long and it's not causal. Not only does it have infinite length, but it also decays only very slowly with time, so you typically need a large number of samples to get a decent accuracy.
This, in turn, results in long latency, high computational cost and fairly large "transition" areas at the beginning and end of the output.
$endgroup$
Sinc() interpolation looks nice on paper or in text books but in practice it's rarely a good solution. The main problem is that the sinc() impulse response is infinitely long and it's not causal. Not only does it have infinite length, but it also decays only very slowly with time, so you typically need a large number of samples to get a decent accuracy.
This, in turn, results in long latency, high computational cost and fairly large "transition" areas at the beginning and end of the output.
answered 7 hours ago
HilmarHilmar
11.7k1 gold badge12 silver badges18 bronze badges
11.7k1 gold badge12 silver badges18 bronze badges
$begingroup$
i think, in practice, a Kaiser-windowed sinc() interpolation can be very good. nearly always, not rarely. nearly as good as Parks-McClellan (firpm()
) or Least Squares (firls()
) and sometimes better, if you compare apples to apples. also sometimesfirpm()
chokes for kernels bigger than 2048 andfirls()
chokes for kernels bigger than 4096. but the kernal size is the product of the number of phases (sometimes i like that as high as 512) and the number of FIR taps (like 32 or 64). but you can make it as big as you want withkaiser()
andsinc()
.
$endgroup$
– robert bristow-johnson
6 hours ago
$begingroup$
so the choice by @Fat32 range of -32 to 32 is arbitrary ?
$endgroup$
– Machupicchu
6 hours ago
$begingroup$
not arbitrary, it's just that we know from FIR filter design that we will need about 32 taps (which means you're looking at 16 samples on the left and 16 samples on the right) to do a decent job of high-quality interpolation of audio. but to do a really good job of it, you might need 64 taps. the number of FIR taps times the number of phases in the polyphase FIR filter is the total length of the the FIR that you would need to design using tools likefirpm()
orfirls()
in MATLAB.
$endgroup$
– robert bristow-johnson
2 hours ago
add a comment |
$begingroup$
i think, in practice, a Kaiser-windowed sinc() interpolation can be very good. nearly always, not rarely. nearly as good as Parks-McClellan (firpm()
) or Least Squares (firls()
) and sometimes better, if you compare apples to apples. also sometimesfirpm()
chokes for kernels bigger than 2048 andfirls()
chokes for kernels bigger than 4096. but the kernal size is the product of the number of phases (sometimes i like that as high as 512) and the number of FIR taps (like 32 or 64). but you can make it as big as you want withkaiser()
andsinc()
.
$endgroup$
– robert bristow-johnson
6 hours ago
$begingroup$
so the choice by @Fat32 range of -32 to 32 is arbitrary ?
$endgroup$
– Machupicchu
6 hours ago
$begingroup$
not arbitrary, it's just that we know from FIR filter design that we will need about 32 taps (which means you're looking at 16 samples on the left and 16 samples on the right) to do a decent job of high-quality interpolation of audio. but to do a really good job of it, you might need 64 taps. the number of FIR taps times the number of phases in the polyphase FIR filter is the total length of the the FIR that you would need to design using tools likefirpm()
orfirls()
in MATLAB.
$endgroup$
– robert bristow-johnson
2 hours ago
$begingroup$
i think, in practice, a Kaiser-windowed sinc() interpolation can be very good. nearly always, not rarely. nearly as good as Parks-McClellan (
firpm()
) or Least Squares (firls()
) and sometimes better, if you compare apples to apples. also sometimes firpm()
chokes for kernels bigger than 2048 and firls()
chokes for kernels bigger than 4096. but the kernal size is the product of the number of phases (sometimes i like that as high as 512) and the number of FIR taps (like 32 or 64). but you can make it as big as you want with kaiser()
and sinc()
.$endgroup$
– robert bristow-johnson
6 hours ago
$begingroup$
i think, in practice, a Kaiser-windowed sinc() interpolation can be very good. nearly always, not rarely. nearly as good as Parks-McClellan (
firpm()
) or Least Squares (firls()
) and sometimes better, if you compare apples to apples. also sometimes firpm()
chokes for kernels bigger than 2048 and firls()
chokes for kernels bigger than 4096. but the kernal size is the product of the number of phases (sometimes i like that as high as 512) and the number of FIR taps (like 32 or 64). but you can make it as big as you want with kaiser()
and sinc()
.$endgroup$
– robert bristow-johnson
6 hours ago
$begingroup$
so the choice by @Fat32 range of -32 to 32 is arbitrary ?
$endgroup$
– Machupicchu
6 hours ago
$begingroup$
so the choice by @Fat32 range of -32 to 32 is arbitrary ?
$endgroup$
– Machupicchu
6 hours ago
$begingroup$
not arbitrary, it's just that we know from FIR filter design that we will need about 32 taps (which means you're looking at 16 samples on the left and 16 samples on the right) to do a decent job of high-quality interpolation of audio. but to do a really good job of it, you might need 64 taps. the number of FIR taps times the number of phases in the polyphase FIR filter is the total length of the the FIR that you would need to design using tools like
firpm()
or firls()
in MATLAB.$endgroup$
– robert bristow-johnson
2 hours ago
$begingroup$
not arbitrary, it's just that we know from FIR filter design that we will need about 32 taps (which means you're looking at 16 samples on the left and 16 samples on the right) to do a decent job of high-quality interpolation of audio. but to do a really good job of it, you might need 64 taps. the number of FIR taps times the number of phases in the polyphase FIR filter is the total length of the the FIR that you would need to design using tools like
firpm()
or firls()
in MATLAB.$endgroup$
– robert bristow-johnson
2 hours ago
add a comment |
Thanks for contributing an answer to Signal Processing Stack Exchange!
- Please be sure to answer the question. Provide details and share your research!
But avoid …
- Asking for help, clarification, or responding to other answers.
- Making statements based on opinion; back them up with references or personal experience.
Use MathJax to format equations. MathJax reference.
To learn more, see our tips on writing great answers.
Sign up or log in
StackExchange.ready(function ()
StackExchange.helpers.onClickDraftSave('#login-link');
);
Sign up using Google
Sign up using Facebook
Sign up using Email and Password
Post as a guest
Required, but never shown
StackExchange.ready(
function ()
StackExchange.openid.initPostLogin('.new-post-login', 'https%3a%2f%2fdsp.stackexchange.com%2fquestions%2f60179%2fsinc-interpolation-in-spatial-domain%23new-answer', 'question_page');
);
Post as a guest
Required, but never shown
Sign up or log in
StackExchange.ready(function ()
StackExchange.helpers.onClickDraftSave('#login-link');
);
Sign up using Google
Sign up using Facebook
Sign up using Email and Password
Post as a guest
Required, but never shown
Sign up or log in
StackExchange.ready(function ()
StackExchange.helpers.onClickDraftSave('#login-link');
);
Sign up using Google
Sign up using Facebook
Sign up using Email and Password
Post as a guest
Required, but never shown
Sign up or log in
StackExchange.ready(function ()
StackExchange.helpers.onClickDraftSave('#login-link');
);
Sign up using Google
Sign up using Facebook
Sign up using Email and Password
Sign up using Google
Sign up using Facebook
Sign up using Email and Password
Post as a guest
Required, but never shown
Required, but never shown
Required, but never shown
Required, but never shown
Required, but never shown
Required, but never shown
Required, but never shown
Required, but never shown
Required, but never shown
jOQ4BzSOR,KJVgVwb
$begingroup$
please help! :)
$endgroup$
– Machupicchu
10 hours ago