Do Bayesian credible intervals treat the estimated parameter as a random variable?From a Bayesian probability perspective, why doesn't a 95% confidence interval contain the true parameter with 95% probability?How is data generated in the Bayesian framework and what is the nature on the parameter that generates the data?Mathematical proof that the posterior probability that a CI contains the true parameter is in $0,1$Is It Ever Appropriate to Treat a Bayesian Credible Interval as a Frequentist Confidence Interval?Is bias a frequentist concept or a Bayesian concept?How to use simulation to check the correctness of my Bayesian model?Interpretation of confidence interval in Bayesian terms
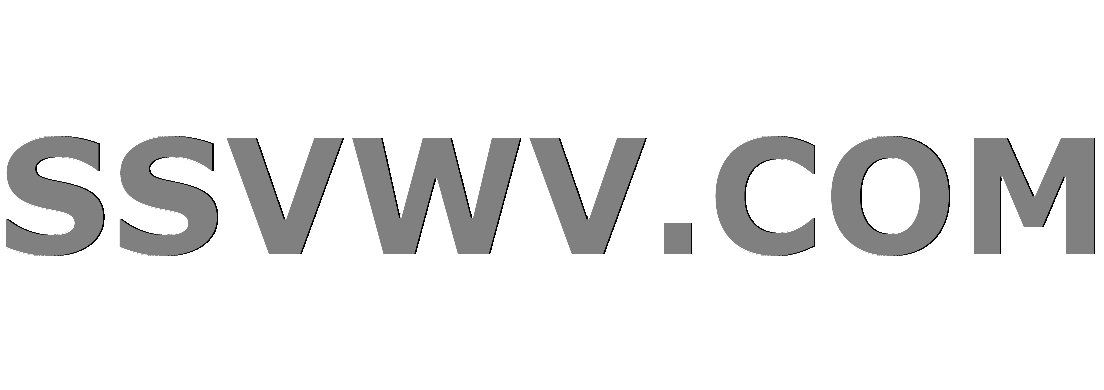
Multi tool use
Asymmetric table
Tex Quotes(UVa 272)
Duplicate Files
Why in most German places is the church the tallest building?
Was there ever a treaty between 2 entities with significantly different translations to the detriment of one party?
How to find out the average duration of the peer-review process for a given journal?
Where was Carl Sagan working on a plan to detonate a nuke on the Moon? Where was he applying when he leaked it?
What does zitch dog mean?
Why is 1. d4 Nf6 2. c4 e6 3. Bg5 almost never played?
What verb is かまされる?
Rent contract say that pets are not allowed. Possible repercussions if bringing the pet anyway?
What is the difference between "Grippe" and "Männergrippe"?
Notepad++ cannot print
Lost property on Portuguese trains
How do thermal tapes transfer heat despite their low thermal conductivity?
What is the best type of paint to paint a shipping container?
Are the A380 engines interchangeable (given they are not all equipped with reverse)?
How can I unambiguously ask for a new user's "Display Name"?
Do they have Supervillain(s)?
How many String objects would be created when concatenating multiple Strings?
Heyacrazy: No Diagonals
Disambiguation of "nobis vobis" and "nobis nobis"
Are there any elected officials in the U.S. who are not legislators, judges, or constitutional officers?
Duplicate instruments in unison in an orchestra
Do Bayesian credible intervals treat the estimated parameter as a random variable?
From a Bayesian probability perspective, why doesn't a 95% confidence interval contain the true parameter with 95% probability?How is data generated in the Bayesian framework and what is the nature on the parameter that generates the data?Mathematical proof that the posterior probability that a CI contains the true parameter is in $0,1$Is It Ever Appropriate to Treat a Bayesian Credible Interval as a Frequentist Confidence Interval?Is bias a frequentist concept or a Bayesian concept?How to use simulation to check the correctness of my Bayesian model?Interpretation of confidence interval in Bayesian terms
.everyoneloves__top-leaderboard:empty,.everyoneloves__mid-leaderboard:empty,.everyoneloves__bot-mid-leaderboard:empty margin-bottom:0;
$begingroup$
I read the following paragraph on Wikipedia recently:
Bayesian intervals treat their bounds as fixed and the estimated parameter as a random variable, whereas frequentist confidence intervals treat their bounds as random variables and the parameter as a fixed value.
However, I am not sure whether this is true. My interpretation of the credible interval was that it encapsulated our own uncertainty about the true value of the estimated parameter but that the estimated parameter itself did have some kind of 'true' value.
This is slightly different to saying that the estimated parameter is a 'random variable'. Am I wrong?
bayesian empirical-bayes
$endgroup$
add a comment |
$begingroup$
I read the following paragraph on Wikipedia recently:
Bayesian intervals treat their bounds as fixed and the estimated parameter as a random variable, whereas frequentist confidence intervals treat their bounds as random variables and the parameter as a fixed value.
However, I am not sure whether this is true. My interpretation of the credible interval was that it encapsulated our own uncertainty about the true value of the estimated parameter but that the estimated parameter itself did have some kind of 'true' value.
This is slightly different to saying that the estimated parameter is a 'random variable'. Am I wrong?
bayesian empirical-bayes
$endgroup$
$begingroup$
I would not defend every word choice, but the Wikipedia quote is essentially correct. Bayesian inference begins with a prior probability distribution on the parameter, taken to be a random variable.
$endgroup$
– BruceET
6 hours ago
$begingroup$
The sentence is confusing. In a Bayesian perspective, the parameter $theta$ is treated as random, while the estimator of the parameter $hattheta(x)$ is not. What is the estimated parameter?
$endgroup$
– Xi'an
5 hours ago
$begingroup$
I agree that it is confusing. To take an example consider the simple beta-binomial model. My question is: how do we interpret the posterior beta distribution of the parameter 'p'? Are we saying that it reflects the fact that 'p' itself is literally a random variable or does it reflect our own uncertainty about what 'p' could be?
$endgroup$
– Johnny Breen
5 hours ago
add a comment |
$begingroup$
I read the following paragraph on Wikipedia recently:
Bayesian intervals treat their bounds as fixed and the estimated parameter as a random variable, whereas frequentist confidence intervals treat their bounds as random variables and the parameter as a fixed value.
However, I am not sure whether this is true. My interpretation of the credible interval was that it encapsulated our own uncertainty about the true value of the estimated parameter but that the estimated parameter itself did have some kind of 'true' value.
This is slightly different to saying that the estimated parameter is a 'random variable'. Am I wrong?
bayesian empirical-bayes
$endgroup$
I read the following paragraph on Wikipedia recently:
Bayesian intervals treat their bounds as fixed and the estimated parameter as a random variable, whereas frequentist confidence intervals treat their bounds as random variables and the parameter as a fixed value.
However, I am not sure whether this is true. My interpretation of the credible interval was that it encapsulated our own uncertainty about the true value of the estimated parameter but that the estimated parameter itself did have some kind of 'true' value.
This is slightly different to saying that the estimated parameter is a 'random variable'. Am I wrong?
bayesian empirical-bayes
bayesian empirical-bayes
asked 8 hours ago
Johnny BreenJohnny Breen
1263 bronze badges
1263 bronze badges
$begingroup$
I would not defend every word choice, but the Wikipedia quote is essentially correct. Bayesian inference begins with a prior probability distribution on the parameter, taken to be a random variable.
$endgroup$
– BruceET
6 hours ago
$begingroup$
The sentence is confusing. In a Bayesian perspective, the parameter $theta$ is treated as random, while the estimator of the parameter $hattheta(x)$ is not. What is the estimated parameter?
$endgroup$
– Xi'an
5 hours ago
$begingroup$
I agree that it is confusing. To take an example consider the simple beta-binomial model. My question is: how do we interpret the posterior beta distribution of the parameter 'p'? Are we saying that it reflects the fact that 'p' itself is literally a random variable or does it reflect our own uncertainty about what 'p' could be?
$endgroup$
– Johnny Breen
5 hours ago
add a comment |
$begingroup$
I would not defend every word choice, but the Wikipedia quote is essentially correct. Bayesian inference begins with a prior probability distribution on the parameter, taken to be a random variable.
$endgroup$
– BruceET
6 hours ago
$begingroup$
The sentence is confusing. In a Bayesian perspective, the parameter $theta$ is treated as random, while the estimator of the parameter $hattheta(x)$ is not. What is the estimated parameter?
$endgroup$
– Xi'an
5 hours ago
$begingroup$
I agree that it is confusing. To take an example consider the simple beta-binomial model. My question is: how do we interpret the posterior beta distribution of the parameter 'p'? Are we saying that it reflects the fact that 'p' itself is literally a random variable or does it reflect our own uncertainty about what 'p' could be?
$endgroup$
– Johnny Breen
5 hours ago
$begingroup$
I would not defend every word choice, but the Wikipedia quote is essentially correct. Bayesian inference begins with a prior probability distribution on the parameter, taken to be a random variable.
$endgroup$
– BruceET
6 hours ago
$begingroup$
I would not defend every word choice, but the Wikipedia quote is essentially correct. Bayesian inference begins with a prior probability distribution on the parameter, taken to be a random variable.
$endgroup$
– BruceET
6 hours ago
$begingroup$
The sentence is confusing. In a Bayesian perspective, the parameter $theta$ is treated as random, while the estimator of the parameter $hattheta(x)$ is not. What is the estimated parameter?
$endgroup$
– Xi'an
5 hours ago
$begingroup$
The sentence is confusing. In a Bayesian perspective, the parameter $theta$ is treated as random, while the estimator of the parameter $hattheta(x)$ is not. What is the estimated parameter?
$endgroup$
– Xi'an
5 hours ago
$begingroup$
I agree that it is confusing. To take an example consider the simple beta-binomial model. My question is: how do we interpret the posterior beta distribution of the parameter 'p'? Are we saying that it reflects the fact that 'p' itself is literally a random variable or does it reflect our own uncertainty about what 'p' could be?
$endgroup$
– Johnny Breen
5 hours ago
$begingroup$
I agree that it is confusing. To take an example consider the simple beta-binomial model. My question is: how do we interpret the posterior beta distribution of the parameter 'p'? Are we saying that it reflects the fact that 'p' itself is literally a random variable or does it reflect our own uncertainty about what 'p' could be?
$endgroup$
– Johnny Breen
5 hours ago
add a comment |
2 Answers
2
active
oldest
votes
$begingroup$
Consider the situation in which you have $n = 20$ observations of a binary (2-coutcome) process. Often the two possible outcomes on each trial are called Success and Failure.
Frequentist confidence interval. Suppose you observe $x = 15$ successes in the $n = 20$ trials. View the number $X$ of Successes as a random variable $X sim mathsfBinom(n=20; p),$ where the success probability $p$ is an unknown constant. The Wald 95% frequentist confidence interval
is based on $hat p = 15/20 = 0.75,$ an estimate of $p.$
Using a normal approximation, this CI is of the form $hat p pm 1.96sqrthat p(1-hat p)/n$ or
$(0.560, 0.940).$ [The somewhat improved Agresti-Coull
style of 95% CI is $(0.526, 0.890).]$
A common interpretation is that the procedure that
produces such an interval will produce lower and upper confidence limits that include the true value of $p$ in 95% of instances over the long run. [The advantage of the Agresti-Coull interval is that the long run proportion of such inclusions is nearer to 95% than for the Wald interval.]
Bayesian credible interval. The Bayesian approach
begins by treating $p$ as a random variable. Prior to seeing data, if we have no prior experience with the kind binomial experiment being conducted or no personal
opinion as to the distribution of $p,$ we may choose
the 'flat' or 'noninformative' uniform distribution,
saying $p sim mathsfUnif(0, 1) equiv
mathsfBeta(1,1).$
Then, given 15 successes in 20 binomial trials, we find the posterior distribution of $p$ as
the product of the prior distribution and the binomial likelihood function.
$$f(p|x) propto p^1-1(1-p)^1-1 times
p^15(1-p)^5 propto
p^16-1(1-p)^6-1,$$
where the symbol $propto$ (read 'proportional to')
indicates that we are omitting 'norming' constant
factors of the distributions, which do not contain $p.$
Without the norming factor, a density function or PMF
is called the 'kernel' of the distribution.
Here we recognize that the kernel of the posterior distribution is that of the distribution $mathsfBeta(16, 6).$ Then a 95% Bayesian posterior interval
or credible interval is found by cutting 2.5% from each tail of the posterior distribution. Here is the result from R:
$(0.528,0.887).$ [For information about beta distributions, see Wikipedia.]
qbeta(c(.025,.975), 16, 6)
[1] 0.5283402 0.8871906
If we believed the prior to be reasonable and believe that
the 20-trial binomial experiment was fairly conducted,
then logically we must expect the Bayesian
interval estimate to give useful information about
the experiment at hand---with no reference to a hypothetical long-run future.
Notice that this Bayesian credible interval
is numerically similar to the Agresti-Coull confidence interval. However, as you point out,
the interpretations of the two types of interval estimates (frequentist and Bayesian) are not the same.
Informative prior. Before we saw the data, if we had reason to believe
that $p approx 2/3,$ then we might have chosen the
distribution $mathsfBeta(8,4)$ as the prior distribution. [This distribution has mean 2/3, standard deviation about 0.35, and puts about 95% of its
probability in the interval $(0.39, 0.89).$]
qbeta(c(.025,.975), 8,4)
[1] 0.3902574 0.8907366
In that case, multiplying the prior by the likelihood gives the posterior kernel of $mathsfBeta(23,7),$
so that the 95% Bayesian credible interval is
$(0.603, 0.897).$ The posterior distribution is a melding of the information in the prior and the likelihood, which are in rough agreement, so the resulting Bayesian interval
estimate is shorter than than the interval from
the flat prior.
qbeta(c(.025,.975), 23,7)
[1] 0.6027531 0.8970164
Notes: (1) The beta prior and binomial likelihood function
are 'conjugage`, that is, mathematically compatible in a way that allows us to find the posterior distribution without computation. Sometimes, there does not seem to
be a prior distribution that is conjugate with the likelihood. The it may be necessary to use numerical integration to find the posterior distribution.
(2) A Bayesian credible interval from an noninformative prior essentially depends on the likelihood function. Also, much of frequentist inference depends of the likelihood function. Thus is is not
a surprise that a Bayesian credible interval from a flat prior may be numerically similar to a frequentist confidence interval based on the same likelihood.
$endgroup$
add a comment |
$begingroup$
Your interpretation is correct. In my opinion that particular passage in the Wikipedia article obfuscates a simple concept with opaque technical language. The initial passage is much clearer: "is an interval within which an unobserved parameter value falls with a particular subjective probability".
The technical term "random variable" is misleading, especially from a Bayesian point of view. It's still used just out of tradition; take a look at Shafer's intriguing historical study When to call a variable random about its origins. From a Bayesian point of view, "random" simply means "unknown" or "uncertain" (for whatever reason), and "variable" is a misnomer for "quantity" or "value". For example, when we try to assess our uncertainty about the speed of light $c$ from a measurement or experiment, we speak of $c$ as a "random variable"; but it's obviously not "random" (and what does "random" mean?), nor is it "variable" – in fact, it's a constant. It's just a physical constant whose exact value we're uncertain about.
See § 16.4 (and other places) in Jaynes's book for an illuminating discussion of this topic.
In frequentist theory the term "random variable" may have a different meaning though. I'm not an expert in this theory, so I won't try to define it there. I think there's some literature around that shows that frequentist confidence intervals and Bayesian intervals can be quite different; see for example Confidence intervals vs Bayesian intervals or https://www.ncbi.nlm.nih.gov/pubmed/6830080.
$endgroup$
$begingroup$
(+1) Jaynes has a lot to say that is important, but I think that the linked paper Confidence Intervals vs Bayesian Intervals is largely a polemic, and it may have been more relevant in the past when Bayesian methods were less accepted.
$endgroup$
– Michael Lew
2 hours ago
add a comment |
Your Answer
StackExchange.ready(function()
var channelOptions =
tags: "".split(" "),
id: "65"
;
initTagRenderer("".split(" "), "".split(" "), channelOptions);
StackExchange.using("externalEditor", function()
// Have to fire editor after snippets, if snippets enabled
if (StackExchange.settings.snippets.snippetsEnabled)
StackExchange.using("snippets", function()
createEditor();
);
else
createEditor();
);
function createEditor()
StackExchange.prepareEditor(
heartbeatType: 'answer',
autoActivateHeartbeat: false,
convertImagesToLinks: false,
noModals: true,
showLowRepImageUploadWarning: true,
reputationToPostImages: null,
bindNavPrevention: true,
postfix: "",
imageUploader:
brandingHtml: "Powered by u003ca class="icon-imgur-white" href="https://imgur.com/"u003eu003c/au003e",
contentPolicyHtml: "User contributions licensed under u003ca href="https://creativecommons.org/licenses/by-sa/3.0/"u003ecc by-sa 3.0 with attribution requiredu003c/au003e u003ca href="https://stackoverflow.com/legal/content-policy"u003e(content policy)u003c/au003e",
allowUrls: true
,
onDemand: true,
discardSelector: ".discard-answer"
,immediatelyShowMarkdownHelp:true
);
);
Sign up or log in
StackExchange.ready(function ()
StackExchange.helpers.onClickDraftSave('#login-link');
);
Sign up using Google
Sign up using Facebook
Sign up using Email and Password
Post as a guest
Required, but never shown
StackExchange.ready(
function ()
StackExchange.openid.initPostLogin('.new-post-login', 'https%3a%2f%2fstats.stackexchange.com%2fquestions%2f423548%2fdo-bayesian-credible-intervals-treat-the-estimated-parameter-as-a-random-variabl%23new-answer', 'question_page');
);
Post as a guest
Required, but never shown
2 Answers
2
active
oldest
votes
2 Answers
2
active
oldest
votes
active
oldest
votes
active
oldest
votes
$begingroup$
Consider the situation in which you have $n = 20$ observations of a binary (2-coutcome) process. Often the two possible outcomes on each trial are called Success and Failure.
Frequentist confidence interval. Suppose you observe $x = 15$ successes in the $n = 20$ trials. View the number $X$ of Successes as a random variable $X sim mathsfBinom(n=20; p),$ where the success probability $p$ is an unknown constant. The Wald 95% frequentist confidence interval
is based on $hat p = 15/20 = 0.75,$ an estimate of $p.$
Using a normal approximation, this CI is of the form $hat p pm 1.96sqrthat p(1-hat p)/n$ or
$(0.560, 0.940).$ [The somewhat improved Agresti-Coull
style of 95% CI is $(0.526, 0.890).]$
A common interpretation is that the procedure that
produces such an interval will produce lower and upper confidence limits that include the true value of $p$ in 95% of instances over the long run. [The advantage of the Agresti-Coull interval is that the long run proportion of such inclusions is nearer to 95% than for the Wald interval.]
Bayesian credible interval. The Bayesian approach
begins by treating $p$ as a random variable. Prior to seeing data, if we have no prior experience with the kind binomial experiment being conducted or no personal
opinion as to the distribution of $p,$ we may choose
the 'flat' or 'noninformative' uniform distribution,
saying $p sim mathsfUnif(0, 1) equiv
mathsfBeta(1,1).$
Then, given 15 successes in 20 binomial trials, we find the posterior distribution of $p$ as
the product of the prior distribution and the binomial likelihood function.
$$f(p|x) propto p^1-1(1-p)^1-1 times
p^15(1-p)^5 propto
p^16-1(1-p)^6-1,$$
where the symbol $propto$ (read 'proportional to')
indicates that we are omitting 'norming' constant
factors of the distributions, which do not contain $p.$
Without the norming factor, a density function or PMF
is called the 'kernel' of the distribution.
Here we recognize that the kernel of the posterior distribution is that of the distribution $mathsfBeta(16, 6).$ Then a 95% Bayesian posterior interval
or credible interval is found by cutting 2.5% from each tail of the posterior distribution. Here is the result from R:
$(0.528,0.887).$ [For information about beta distributions, see Wikipedia.]
qbeta(c(.025,.975), 16, 6)
[1] 0.5283402 0.8871906
If we believed the prior to be reasonable and believe that
the 20-trial binomial experiment was fairly conducted,
then logically we must expect the Bayesian
interval estimate to give useful information about
the experiment at hand---with no reference to a hypothetical long-run future.
Notice that this Bayesian credible interval
is numerically similar to the Agresti-Coull confidence interval. However, as you point out,
the interpretations of the two types of interval estimates (frequentist and Bayesian) are not the same.
Informative prior. Before we saw the data, if we had reason to believe
that $p approx 2/3,$ then we might have chosen the
distribution $mathsfBeta(8,4)$ as the prior distribution. [This distribution has mean 2/3, standard deviation about 0.35, and puts about 95% of its
probability in the interval $(0.39, 0.89).$]
qbeta(c(.025,.975), 8,4)
[1] 0.3902574 0.8907366
In that case, multiplying the prior by the likelihood gives the posterior kernel of $mathsfBeta(23,7),$
so that the 95% Bayesian credible interval is
$(0.603, 0.897).$ The posterior distribution is a melding of the information in the prior and the likelihood, which are in rough agreement, so the resulting Bayesian interval
estimate is shorter than than the interval from
the flat prior.
qbeta(c(.025,.975), 23,7)
[1] 0.6027531 0.8970164
Notes: (1) The beta prior and binomial likelihood function
are 'conjugage`, that is, mathematically compatible in a way that allows us to find the posterior distribution without computation. Sometimes, there does not seem to
be a prior distribution that is conjugate with the likelihood. The it may be necessary to use numerical integration to find the posterior distribution.
(2) A Bayesian credible interval from an noninformative prior essentially depends on the likelihood function. Also, much of frequentist inference depends of the likelihood function. Thus is is not
a surprise that a Bayesian credible interval from a flat prior may be numerically similar to a frequentist confidence interval based on the same likelihood.
$endgroup$
add a comment |
$begingroup$
Consider the situation in which you have $n = 20$ observations of a binary (2-coutcome) process. Often the two possible outcomes on each trial are called Success and Failure.
Frequentist confidence interval. Suppose you observe $x = 15$ successes in the $n = 20$ trials. View the number $X$ of Successes as a random variable $X sim mathsfBinom(n=20; p),$ where the success probability $p$ is an unknown constant. The Wald 95% frequentist confidence interval
is based on $hat p = 15/20 = 0.75,$ an estimate of $p.$
Using a normal approximation, this CI is of the form $hat p pm 1.96sqrthat p(1-hat p)/n$ or
$(0.560, 0.940).$ [The somewhat improved Agresti-Coull
style of 95% CI is $(0.526, 0.890).]$
A common interpretation is that the procedure that
produces such an interval will produce lower and upper confidence limits that include the true value of $p$ in 95% of instances over the long run. [The advantage of the Agresti-Coull interval is that the long run proportion of such inclusions is nearer to 95% than for the Wald interval.]
Bayesian credible interval. The Bayesian approach
begins by treating $p$ as a random variable. Prior to seeing data, if we have no prior experience with the kind binomial experiment being conducted or no personal
opinion as to the distribution of $p,$ we may choose
the 'flat' or 'noninformative' uniform distribution,
saying $p sim mathsfUnif(0, 1) equiv
mathsfBeta(1,1).$
Then, given 15 successes in 20 binomial trials, we find the posterior distribution of $p$ as
the product of the prior distribution and the binomial likelihood function.
$$f(p|x) propto p^1-1(1-p)^1-1 times
p^15(1-p)^5 propto
p^16-1(1-p)^6-1,$$
where the symbol $propto$ (read 'proportional to')
indicates that we are omitting 'norming' constant
factors of the distributions, which do not contain $p.$
Without the norming factor, a density function or PMF
is called the 'kernel' of the distribution.
Here we recognize that the kernel of the posterior distribution is that of the distribution $mathsfBeta(16, 6).$ Then a 95% Bayesian posterior interval
or credible interval is found by cutting 2.5% from each tail of the posterior distribution. Here is the result from R:
$(0.528,0.887).$ [For information about beta distributions, see Wikipedia.]
qbeta(c(.025,.975), 16, 6)
[1] 0.5283402 0.8871906
If we believed the prior to be reasonable and believe that
the 20-trial binomial experiment was fairly conducted,
then logically we must expect the Bayesian
interval estimate to give useful information about
the experiment at hand---with no reference to a hypothetical long-run future.
Notice that this Bayesian credible interval
is numerically similar to the Agresti-Coull confidence interval. However, as you point out,
the interpretations of the two types of interval estimates (frequentist and Bayesian) are not the same.
Informative prior. Before we saw the data, if we had reason to believe
that $p approx 2/3,$ then we might have chosen the
distribution $mathsfBeta(8,4)$ as the prior distribution. [This distribution has mean 2/3, standard deviation about 0.35, and puts about 95% of its
probability in the interval $(0.39, 0.89).$]
qbeta(c(.025,.975), 8,4)
[1] 0.3902574 0.8907366
In that case, multiplying the prior by the likelihood gives the posterior kernel of $mathsfBeta(23,7),$
so that the 95% Bayesian credible interval is
$(0.603, 0.897).$ The posterior distribution is a melding of the information in the prior and the likelihood, which are in rough agreement, so the resulting Bayesian interval
estimate is shorter than than the interval from
the flat prior.
qbeta(c(.025,.975), 23,7)
[1] 0.6027531 0.8970164
Notes: (1) The beta prior and binomial likelihood function
are 'conjugage`, that is, mathematically compatible in a way that allows us to find the posterior distribution without computation. Sometimes, there does not seem to
be a prior distribution that is conjugate with the likelihood. The it may be necessary to use numerical integration to find the posterior distribution.
(2) A Bayesian credible interval from an noninformative prior essentially depends on the likelihood function. Also, much of frequentist inference depends of the likelihood function. Thus is is not
a surprise that a Bayesian credible interval from a flat prior may be numerically similar to a frequentist confidence interval based on the same likelihood.
$endgroup$
add a comment |
$begingroup$
Consider the situation in which you have $n = 20$ observations of a binary (2-coutcome) process. Often the two possible outcomes on each trial are called Success and Failure.
Frequentist confidence interval. Suppose you observe $x = 15$ successes in the $n = 20$ trials. View the number $X$ of Successes as a random variable $X sim mathsfBinom(n=20; p),$ where the success probability $p$ is an unknown constant. The Wald 95% frequentist confidence interval
is based on $hat p = 15/20 = 0.75,$ an estimate of $p.$
Using a normal approximation, this CI is of the form $hat p pm 1.96sqrthat p(1-hat p)/n$ or
$(0.560, 0.940).$ [The somewhat improved Agresti-Coull
style of 95% CI is $(0.526, 0.890).]$
A common interpretation is that the procedure that
produces such an interval will produce lower and upper confidence limits that include the true value of $p$ in 95% of instances over the long run. [The advantage of the Agresti-Coull interval is that the long run proportion of such inclusions is nearer to 95% than for the Wald interval.]
Bayesian credible interval. The Bayesian approach
begins by treating $p$ as a random variable. Prior to seeing data, if we have no prior experience with the kind binomial experiment being conducted or no personal
opinion as to the distribution of $p,$ we may choose
the 'flat' or 'noninformative' uniform distribution,
saying $p sim mathsfUnif(0, 1) equiv
mathsfBeta(1,1).$
Then, given 15 successes in 20 binomial trials, we find the posterior distribution of $p$ as
the product of the prior distribution and the binomial likelihood function.
$$f(p|x) propto p^1-1(1-p)^1-1 times
p^15(1-p)^5 propto
p^16-1(1-p)^6-1,$$
where the symbol $propto$ (read 'proportional to')
indicates that we are omitting 'norming' constant
factors of the distributions, which do not contain $p.$
Without the norming factor, a density function or PMF
is called the 'kernel' of the distribution.
Here we recognize that the kernel of the posterior distribution is that of the distribution $mathsfBeta(16, 6).$ Then a 95% Bayesian posterior interval
or credible interval is found by cutting 2.5% from each tail of the posterior distribution. Here is the result from R:
$(0.528,0.887).$ [For information about beta distributions, see Wikipedia.]
qbeta(c(.025,.975), 16, 6)
[1] 0.5283402 0.8871906
If we believed the prior to be reasonable and believe that
the 20-trial binomial experiment was fairly conducted,
then logically we must expect the Bayesian
interval estimate to give useful information about
the experiment at hand---with no reference to a hypothetical long-run future.
Notice that this Bayesian credible interval
is numerically similar to the Agresti-Coull confidence interval. However, as you point out,
the interpretations of the two types of interval estimates (frequentist and Bayesian) are not the same.
Informative prior. Before we saw the data, if we had reason to believe
that $p approx 2/3,$ then we might have chosen the
distribution $mathsfBeta(8,4)$ as the prior distribution. [This distribution has mean 2/3, standard deviation about 0.35, and puts about 95% of its
probability in the interval $(0.39, 0.89).$]
qbeta(c(.025,.975), 8,4)
[1] 0.3902574 0.8907366
In that case, multiplying the prior by the likelihood gives the posterior kernel of $mathsfBeta(23,7),$
so that the 95% Bayesian credible interval is
$(0.603, 0.897).$ The posterior distribution is a melding of the information in the prior and the likelihood, which are in rough agreement, so the resulting Bayesian interval
estimate is shorter than than the interval from
the flat prior.
qbeta(c(.025,.975), 23,7)
[1] 0.6027531 0.8970164
Notes: (1) The beta prior and binomial likelihood function
are 'conjugage`, that is, mathematically compatible in a way that allows us to find the posterior distribution without computation. Sometimes, there does not seem to
be a prior distribution that is conjugate with the likelihood. The it may be necessary to use numerical integration to find the posterior distribution.
(2) A Bayesian credible interval from an noninformative prior essentially depends on the likelihood function. Also, much of frequentist inference depends of the likelihood function. Thus is is not
a surprise that a Bayesian credible interval from a flat prior may be numerically similar to a frequentist confidence interval based on the same likelihood.
$endgroup$
Consider the situation in which you have $n = 20$ observations of a binary (2-coutcome) process. Often the two possible outcomes on each trial are called Success and Failure.
Frequentist confidence interval. Suppose you observe $x = 15$ successes in the $n = 20$ trials. View the number $X$ of Successes as a random variable $X sim mathsfBinom(n=20; p),$ where the success probability $p$ is an unknown constant. The Wald 95% frequentist confidence interval
is based on $hat p = 15/20 = 0.75,$ an estimate of $p.$
Using a normal approximation, this CI is of the form $hat p pm 1.96sqrthat p(1-hat p)/n$ or
$(0.560, 0.940).$ [The somewhat improved Agresti-Coull
style of 95% CI is $(0.526, 0.890).]$
A common interpretation is that the procedure that
produces such an interval will produce lower and upper confidence limits that include the true value of $p$ in 95% of instances over the long run. [The advantage of the Agresti-Coull interval is that the long run proportion of such inclusions is nearer to 95% than for the Wald interval.]
Bayesian credible interval. The Bayesian approach
begins by treating $p$ as a random variable. Prior to seeing data, if we have no prior experience with the kind binomial experiment being conducted or no personal
opinion as to the distribution of $p,$ we may choose
the 'flat' or 'noninformative' uniform distribution,
saying $p sim mathsfUnif(0, 1) equiv
mathsfBeta(1,1).$
Then, given 15 successes in 20 binomial trials, we find the posterior distribution of $p$ as
the product of the prior distribution and the binomial likelihood function.
$$f(p|x) propto p^1-1(1-p)^1-1 times
p^15(1-p)^5 propto
p^16-1(1-p)^6-1,$$
where the symbol $propto$ (read 'proportional to')
indicates that we are omitting 'norming' constant
factors of the distributions, which do not contain $p.$
Without the norming factor, a density function or PMF
is called the 'kernel' of the distribution.
Here we recognize that the kernel of the posterior distribution is that of the distribution $mathsfBeta(16, 6).$ Then a 95% Bayesian posterior interval
or credible interval is found by cutting 2.5% from each tail of the posterior distribution. Here is the result from R:
$(0.528,0.887).$ [For information about beta distributions, see Wikipedia.]
qbeta(c(.025,.975), 16, 6)
[1] 0.5283402 0.8871906
If we believed the prior to be reasonable and believe that
the 20-trial binomial experiment was fairly conducted,
then logically we must expect the Bayesian
interval estimate to give useful information about
the experiment at hand---with no reference to a hypothetical long-run future.
Notice that this Bayesian credible interval
is numerically similar to the Agresti-Coull confidence interval. However, as you point out,
the interpretations of the two types of interval estimates (frequentist and Bayesian) are not the same.
Informative prior. Before we saw the data, if we had reason to believe
that $p approx 2/3,$ then we might have chosen the
distribution $mathsfBeta(8,4)$ as the prior distribution. [This distribution has mean 2/3, standard deviation about 0.35, and puts about 95% of its
probability in the interval $(0.39, 0.89).$]
qbeta(c(.025,.975), 8,4)
[1] 0.3902574 0.8907366
In that case, multiplying the prior by the likelihood gives the posterior kernel of $mathsfBeta(23,7),$
so that the 95% Bayesian credible interval is
$(0.603, 0.897).$ The posterior distribution is a melding of the information in the prior and the likelihood, which are in rough agreement, so the resulting Bayesian interval
estimate is shorter than than the interval from
the flat prior.
qbeta(c(.025,.975), 23,7)
[1] 0.6027531 0.8970164
Notes: (1) The beta prior and binomial likelihood function
are 'conjugage`, that is, mathematically compatible in a way that allows us to find the posterior distribution without computation. Sometimes, there does not seem to
be a prior distribution that is conjugate with the likelihood. The it may be necessary to use numerical integration to find the posterior distribution.
(2) A Bayesian credible interval from an noninformative prior essentially depends on the likelihood function. Also, much of frequentist inference depends of the likelihood function. Thus is is not
a surprise that a Bayesian credible interval from a flat prior may be numerically similar to a frequentist confidence interval based on the same likelihood.
edited 4 hours ago
answered 5 hours ago
BruceETBruceET
13.3k1 gold badge9 silver badges26 bronze badges
13.3k1 gold badge9 silver badges26 bronze badges
add a comment |
add a comment |
$begingroup$
Your interpretation is correct. In my opinion that particular passage in the Wikipedia article obfuscates a simple concept with opaque technical language. The initial passage is much clearer: "is an interval within which an unobserved parameter value falls with a particular subjective probability".
The technical term "random variable" is misleading, especially from a Bayesian point of view. It's still used just out of tradition; take a look at Shafer's intriguing historical study When to call a variable random about its origins. From a Bayesian point of view, "random" simply means "unknown" or "uncertain" (for whatever reason), and "variable" is a misnomer for "quantity" or "value". For example, when we try to assess our uncertainty about the speed of light $c$ from a measurement or experiment, we speak of $c$ as a "random variable"; but it's obviously not "random" (and what does "random" mean?), nor is it "variable" – in fact, it's a constant. It's just a physical constant whose exact value we're uncertain about.
See § 16.4 (and other places) in Jaynes's book for an illuminating discussion of this topic.
In frequentist theory the term "random variable" may have a different meaning though. I'm not an expert in this theory, so I won't try to define it there. I think there's some literature around that shows that frequentist confidence intervals and Bayesian intervals can be quite different; see for example Confidence intervals vs Bayesian intervals or https://www.ncbi.nlm.nih.gov/pubmed/6830080.
$endgroup$
$begingroup$
(+1) Jaynes has a lot to say that is important, but I think that the linked paper Confidence Intervals vs Bayesian Intervals is largely a polemic, and it may have been more relevant in the past when Bayesian methods were less accepted.
$endgroup$
– Michael Lew
2 hours ago
add a comment |
$begingroup$
Your interpretation is correct. In my opinion that particular passage in the Wikipedia article obfuscates a simple concept with opaque technical language. The initial passage is much clearer: "is an interval within which an unobserved parameter value falls with a particular subjective probability".
The technical term "random variable" is misleading, especially from a Bayesian point of view. It's still used just out of tradition; take a look at Shafer's intriguing historical study When to call a variable random about its origins. From a Bayesian point of view, "random" simply means "unknown" or "uncertain" (for whatever reason), and "variable" is a misnomer for "quantity" or "value". For example, when we try to assess our uncertainty about the speed of light $c$ from a measurement or experiment, we speak of $c$ as a "random variable"; but it's obviously not "random" (and what does "random" mean?), nor is it "variable" – in fact, it's a constant. It's just a physical constant whose exact value we're uncertain about.
See § 16.4 (and other places) in Jaynes's book for an illuminating discussion of this topic.
In frequentist theory the term "random variable" may have a different meaning though. I'm not an expert in this theory, so I won't try to define it there. I think there's some literature around that shows that frequentist confidence intervals and Bayesian intervals can be quite different; see for example Confidence intervals vs Bayesian intervals or https://www.ncbi.nlm.nih.gov/pubmed/6830080.
$endgroup$
$begingroup$
(+1) Jaynes has a lot to say that is important, but I think that the linked paper Confidence Intervals vs Bayesian Intervals is largely a polemic, and it may have been more relevant in the past when Bayesian methods were less accepted.
$endgroup$
– Michael Lew
2 hours ago
add a comment |
$begingroup$
Your interpretation is correct. In my opinion that particular passage in the Wikipedia article obfuscates a simple concept with opaque technical language. The initial passage is much clearer: "is an interval within which an unobserved parameter value falls with a particular subjective probability".
The technical term "random variable" is misleading, especially from a Bayesian point of view. It's still used just out of tradition; take a look at Shafer's intriguing historical study When to call a variable random about its origins. From a Bayesian point of view, "random" simply means "unknown" or "uncertain" (for whatever reason), and "variable" is a misnomer for "quantity" or "value". For example, when we try to assess our uncertainty about the speed of light $c$ from a measurement or experiment, we speak of $c$ as a "random variable"; but it's obviously not "random" (and what does "random" mean?), nor is it "variable" – in fact, it's a constant. It's just a physical constant whose exact value we're uncertain about.
See § 16.4 (and other places) in Jaynes's book for an illuminating discussion of this topic.
In frequentist theory the term "random variable" may have a different meaning though. I'm not an expert in this theory, so I won't try to define it there. I think there's some literature around that shows that frequentist confidence intervals and Bayesian intervals can be quite different; see for example Confidence intervals vs Bayesian intervals or https://www.ncbi.nlm.nih.gov/pubmed/6830080.
$endgroup$
Your interpretation is correct. In my opinion that particular passage in the Wikipedia article obfuscates a simple concept with opaque technical language. The initial passage is much clearer: "is an interval within which an unobserved parameter value falls with a particular subjective probability".
The technical term "random variable" is misleading, especially from a Bayesian point of view. It's still used just out of tradition; take a look at Shafer's intriguing historical study When to call a variable random about its origins. From a Bayesian point of view, "random" simply means "unknown" or "uncertain" (for whatever reason), and "variable" is a misnomer for "quantity" or "value". For example, when we try to assess our uncertainty about the speed of light $c$ from a measurement or experiment, we speak of $c$ as a "random variable"; but it's obviously not "random" (and what does "random" mean?), nor is it "variable" – in fact, it's a constant. It's just a physical constant whose exact value we're uncertain about.
See § 16.4 (and other places) in Jaynes's book for an illuminating discussion of this topic.
In frequentist theory the term "random variable" may have a different meaning though. I'm not an expert in this theory, so I won't try to define it there. I think there's some literature around that shows that frequentist confidence intervals and Bayesian intervals can be quite different; see for example Confidence intervals vs Bayesian intervals or https://www.ncbi.nlm.nih.gov/pubmed/6830080.
edited 3 hours ago
answered 4 hours ago


pglpmpglpm
4524 silver badges12 bronze badges
4524 silver badges12 bronze badges
$begingroup$
(+1) Jaynes has a lot to say that is important, but I think that the linked paper Confidence Intervals vs Bayesian Intervals is largely a polemic, and it may have been more relevant in the past when Bayesian methods were less accepted.
$endgroup$
– Michael Lew
2 hours ago
add a comment |
$begingroup$
(+1) Jaynes has a lot to say that is important, but I think that the linked paper Confidence Intervals vs Bayesian Intervals is largely a polemic, and it may have been more relevant in the past when Bayesian methods were less accepted.
$endgroup$
– Michael Lew
2 hours ago
$begingroup$
(+1) Jaynes has a lot to say that is important, but I think that the linked paper Confidence Intervals vs Bayesian Intervals is largely a polemic, and it may have been more relevant in the past when Bayesian methods were less accepted.
$endgroup$
– Michael Lew
2 hours ago
$begingroup$
(+1) Jaynes has a lot to say that is important, but I think that the linked paper Confidence Intervals vs Bayesian Intervals is largely a polemic, and it may have been more relevant in the past when Bayesian methods were less accepted.
$endgroup$
– Michael Lew
2 hours ago
add a comment |
Thanks for contributing an answer to Cross Validated!
- Please be sure to answer the question. Provide details and share your research!
But avoid …
- Asking for help, clarification, or responding to other answers.
- Making statements based on opinion; back them up with references or personal experience.
Use MathJax to format equations. MathJax reference.
To learn more, see our tips on writing great answers.
Sign up or log in
StackExchange.ready(function ()
StackExchange.helpers.onClickDraftSave('#login-link');
);
Sign up using Google
Sign up using Facebook
Sign up using Email and Password
Post as a guest
Required, but never shown
StackExchange.ready(
function ()
StackExchange.openid.initPostLogin('.new-post-login', 'https%3a%2f%2fstats.stackexchange.com%2fquestions%2f423548%2fdo-bayesian-credible-intervals-treat-the-estimated-parameter-as-a-random-variabl%23new-answer', 'question_page');
);
Post as a guest
Required, but never shown
Sign up or log in
StackExchange.ready(function ()
StackExchange.helpers.onClickDraftSave('#login-link');
);
Sign up using Google
Sign up using Facebook
Sign up using Email and Password
Post as a guest
Required, but never shown
Sign up or log in
StackExchange.ready(function ()
StackExchange.helpers.onClickDraftSave('#login-link');
);
Sign up using Google
Sign up using Facebook
Sign up using Email and Password
Post as a guest
Required, but never shown
Sign up or log in
StackExchange.ready(function ()
StackExchange.helpers.onClickDraftSave('#login-link');
);
Sign up using Google
Sign up using Facebook
Sign up using Email and Password
Sign up using Google
Sign up using Facebook
Sign up using Email and Password
Post as a guest
Required, but never shown
Required, but never shown
Required, but never shown
Required, but never shown
Required, but never shown
Required, but never shown
Required, but never shown
Required, but never shown
Required, but never shown
QX7 qKK,CS YgYG,C,724i0en 7iWd2cjoqEG,ADQ78MIS8Frt214SZ AaT0c6yenP7vre7vg
$begingroup$
I would not defend every word choice, but the Wikipedia quote is essentially correct. Bayesian inference begins with a prior probability distribution on the parameter, taken to be a random variable.
$endgroup$
– BruceET
6 hours ago
$begingroup$
The sentence is confusing. In a Bayesian perspective, the parameter $theta$ is treated as random, while the estimator of the parameter $hattheta(x)$ is not. What is the estimated parameter?
$endgroup$
– Xi'an
5 hours ago
$begingroup$
I agree that it is confusing. To take an example consider the simple beta-binomial model. My question is: how do we interpret the posterior beta distribution of the parameter 'p'? Are we saying that it reflects the fact that 'p' itself is literally a random variable or does it reflect our own uncertainty about what 'p' could be?
$endgroup$
– Johnny Breen
5 hours ago