What is a Kravchuk transform and how is it related to Fourier transforms?Fourier Transform Time ScalingFourier Transform of exponentialQuestions related to Laplace TransformInverse Fourier Transform problemHow would Fourier and Cosine Transforms responds to summation of cosines with same frequency but different phases?Discreteness and periodicity in Fourier transformSignificance of sequency ordering in Walsh-Hadamard matricesWhat is the difference between Constant-Q Transform and Wavelet Transform and which is betterCalculating fourier transformWhat is the correct order of operations for a 2D Haar wavelet decomposition?
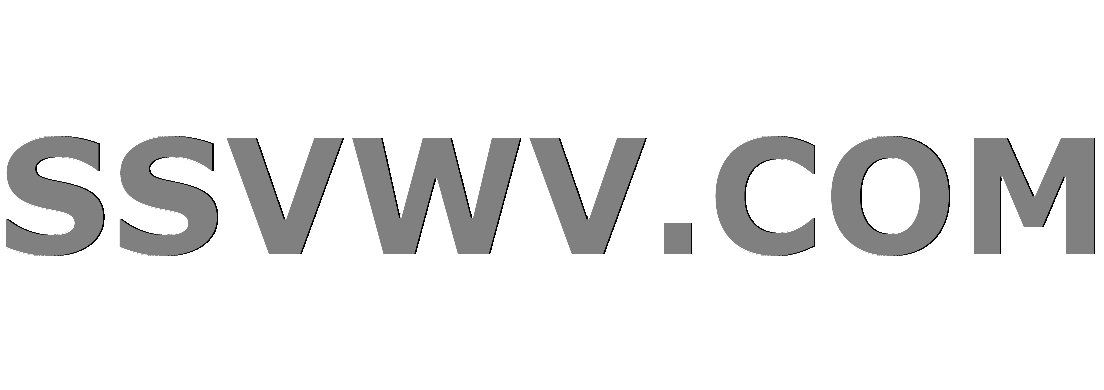
Multi tool use
The most secure way to handle someone forgetting to verify their account?
How should I interpret a promising preprint that was never published in a peer-reviewed journal?
Transistor power dissipation rating
Why teach C using scanf without talking about command line arguments?
Did Hitler say this quote about homeschooling?
Extract the attribute names from a large number of Shapefiles
How important are the Author's mood and feelings for writing a story?
"Je suis petite, moi?", purpose of the "moi"?
When will the last unambiguous evidence of mankind disappear?
Why is the Intel 8086 CPU called a 16-bit CPU?
Evaluate the limit the following series
What's a German word for »Sandbagger«?
Was demon possession only a New Testament phenomenon?
What's the largest an Earth-like planet can be and support Earth's biosphere?
How to decide print orientation?
How to not confuse readers with simultaneous events?
Is encryption still applied if you ignore the SSL certificate warning for self-signed certs?
What makes MOVEQ quicker than a normal MOVE in 68000 assembly?
What is the mistake in this solution?
Why don't humans perceive sound waves as twice the frequency they are?
Somebody hacked my clock
What would be the safest way to drop thousands of small, hard objects from a typical, high wing, GA airplane?
Which family is it?
How was Luke's prosthetic hand in Episode V filmed?
What is a Kravchuk transform and how is it related to Fourier transforms?
Fourier Transform Time ScalingFourier Transform of exponentialQuestions related to Laplace TransformInverse Fourier Transform problemHow would Fourier and Cosine Transforms responds to summation of cosines with same frequency but different phases?Discreteness and periodicity in Fourier transformSignificance of sequency ordering in Walsh-Hadamard matricesWhat is the difference between Constant-Q Transform and Wavelet Transform and which is betterCalculating fourier transformWhat is the correct order of operations for a 2D Haar wavelet decomposition?
.everyoneloves__top-leaderboard:empty,.everyoneloves__mid-leaderboard:empty,.everyoneloves__bot-mid-leaderboard:empty margin-bottom:0;
$begingroup$
It has been stated here : https://phys.org/news/2019-07-quantum-technology.html that the the so-called Kravchuk transform is very important in the field of image processing and possibly in signal processing in general.
I can hardly find any description about this (e.g. not mentioned in Wikipedia, etc.).
It seems to be mentioned in this paper for example:
https://www.researchgate.net/publication/24147178_Mode_analysis_and_signal_restoration_with_Kravchuk_functions
transform
$endgroup$
add a comment |
$begingroup$
It has been stated here : https://phys.org/news/2019-07-quantum-technology.html that the the so-called Kravchuk transform is very important in the field of image processing and possibly in signal processing in general.
I can hardly find any description about this (e.g. not mentioned in Wikipedia, etc.).
It seems to be mentioned in this paper for example:
https://www.researchgate.net/publication/24147178_Mode_analysis_and_signal_restoration_with_Kravchuk_functions
transform
$endgroup$
add a comment |
$begingroup$
It has been stated here : https://phys.org/news/2019-07-quantum-technology.html that the the so-called Kravchuk transform is very important in the field of image processing and possibly in signal processing in general.
I can hardly find any description about this (e.g. not mentioned in Wikipedia, etc.).
It seems to be mentioned in this paper for example:
https://www.researchgate.net/publication/24147178_Mode_analysis_and_signal_restoration_with_Kravchuk_functions
transform
$endgroup$
It has been stated here : https://phys.org/news/2019-07-quantum-technology.html that the the so-called Kravchuk transform is very important in the field of image processing and possibly in signal processing in general.
I can hardly find any description about this (e.g. not mentioned in Wikipedia, etc.).
It seems to be mentioned in this paper for example:
https://www.researchgate.net/publication/24147178_Mode_analysis_and_signal_restoration_with_Kravchuk_functions
transform
transform
asked 8 hours ago
MachupicchuMachupicchu
468 bronze badges
468 bronze badges
add a comment |
add a comment |
1 Answer
1
active
oldest
votes
$begingroup$
Transliterations of Ukrainian names have different avatars. You can find Kravchuk polynomials, and other papers like On Krawtchouk Transforms or Krawtchouk polynomials and Krawtchouk matrices. You can find as well Kravchuk orthogonal polynomials.
As they form an orthogonal basis of polynomials (as well as many others, listed on polpak: Bernoulli, Bernstein, Chebyshev, Hermite, Laguerre, Legendre, Zernike), they are candidates for a transform. Derived moments are used in image processing, and the following paper seems to have a wide audience:
Image analysis by Krawtchouk moments, IEEE Transactions on Image Processing 2003
A new set of orthogonal moments based on the discrete classical
Krawtchouk polynomials is introduced. The Krawtchouk polynomials are
scaled to ensure numerical stability, thus creating a set of weighted
Krawtchouk polynomials. The set of proposed Krawtchouk moments is then
derived from the weighted Krawtchouk polynomials. The orthogonality of
the proposed moments ensures minimal information redundancy. No
numerical approximation is involved in deriving the moments, since the
weighted Krawtchouk polynomials are discrete. These properties make
the Krawtchouk moments well suited as pattern features in the analysis
of two-dimensional images. It is shown that the Krawtchouk moments can
be employed to extract local features of an image, unlike other
orthogonal moments, which generally capture the global features. The
computational aspects of the moments using the recursive and symmetry
properties are discussed. The theoretical framework is validated by an
experiment on image reconstruction using Krawtchouk moments and the
results are compared to that of Zernike, pseudo-Zernike, Legendre, and
Tchebyscheff moments. Krawtchouk moment invariants are constructed
using a linear combination of geometric moment invariants; an object
recognition experiment shows Krawtchouk moment invariants perform
significantly better than Hu's moment invariants in both noise-free
and noisy conditions.
Later, you can read:
Image Analysis Using Hahn Moments, IEEE Transactions on Pattern Analysis and Machine, 2007, where Hahn moments generalize Chebyshev and Krawtchouk moments:
This paper shows how Hahn moments provide a unified understanding of
the recently introduced Chebyshev and Krawtchouk moments. The two
latter moments can be obtained as particular cases of Hahn moments
with the appropriate parameter settings and this fact implies that
Hahn moments encompass all their properties. The aim of this paper is
twofold: (1) To show how Hahn moments, as a generalization of
Chebyshev and Krawtchouk moments, can be used for global and local
feature extraction and (2) to show how Hahn moments can be
incorporated into the framework of normalized convolution to analyze
local structures of irregularly sampled signals.
In Wikipedia's Discrete Fourier transform we find:
The choice of eigenvectors of the DFT matrix has become important in
recent years in order to define a discrete analogue of the fractional
Fourier transform—the DFT matrix can be taken to fractional powers by
exponentiating the eigenvalues (e.g., Rubio and Santhanam, 2005). For
the continuous Fourier transform, the natural orthogonal
eigenfunctions are the Hermite functions, so various discrete
analogues of these have been employed as the eigenvectors of the DFT,
such as the Kravchuk polynomials (Atakishiyev and Wolf, 1997). The
"best" choice of eigenvectors to define a fractional discrete Fourier
transform remains an open question, however.
$endgroup$
$begingroup$
Can we said that "Kravchouk transform" usually is a "fractional Fourier transform"?
$endgroup$
– Machupicchu
8 hours ago
$begingroup$
There are ambiguities on what people call "fractional Fourier transforms", and I am not a practitioner of the Fractional Fourier–Kravchuk transform, but I doubt there is a one-to-one correspondance
$endgroup$
– Laurent Duval
7 hours ago
1
$begingroup$
ah ok. It seems strange that they cite this obscure Kravchouk in phys.org/news/2019-07-quantum-technology.html as it does not seem to be known by many DSP people ?
$endgroup$
– Machupicchu
7 hours ago
1
$begingroup$
oh ok. So you would say it is "overstated" as important?
$endgroup$
– Machupicchu
7 hours ago
1
$begingroup$
This is another area where keeping the distinction and relationship between the discrete and continuous case is important. I like to use Legendre polynomials. I tend to think of them as "the orthogonal Taylor series", however, the discrete version in not orthogonal. You need to apply something like Gram-Schmidt (G-S) to rectify that for discrete usage. Then keeping the same N becomes important.
$endgroup$
– Cedron Dawg
7 hours ago
|
show 2 more comments
Your Answer
StackExchange.ready(function()
var channelOptions =
tags: "".split(" "),
id: "295"
;
initTagRenderer("".split(" "), "".split(" "), channelOptions);
StackExchange.using("externalEditor", function()
// Have to fire editor after snippets, if snippets enabled
if (StackExchange.settings.snippets.snippetsEnabled)
StackExchange.using("snippets", function()
createEditor();
);
else
createEditor();
);
function createEditor()
StackExchange.prepareEditor(
heartbeatType: 'answer',
autoActivateHeartbeat: false,
convertImagesToLinks: false,
noModals: true,
showLowRepImageUploadWarning: true,
reputationToPostImages: null,
bindNavPrevention: true,
postfix: "",
imageUploader:
brandingHtml: "Powered by u003ca class="icon-imgur-white" href="https://imgur.com/"u003eu003c/au003e",
contentPolicyHtml: "User contributions licensed under u003ca href="https://creativecommons.org/licenses/by-sa/3.0/"u003ecc by-sa 3.0 with attribution requiredu003c/au003e u003ca href="https://stackoverflow.com/legal/content-policy"u003e(content policy)u003c/au003e",
allowUrls: true
,
noCode: true, onDemand: true,
discardSelector: ".discard-answer"
,immediatelyShowMarkdownHelp:true
);
);
Sign up or log in
StackExchange.ready(function ()
StackExchange.helpers.onClickDraftSave('#login-link');
);
Sign up using Google
Sign up using Facebook
Sign up using Email and Password
Post as a guest
Required, but never shown
StackExchange.ready(
function ()
StackExchange.openid.initPostLogin('.new-post-login', 'https%3a%2f%2fdsp.stackexchange.com%2fquestions%2f59657%2fwhat-is-a-kravchuk-transform-and-how-is-it-related-to-fourier-transforms%23new-answer', 'question_page');
);
Post as a guest
Required, but never shown
1 Answer
1
active
oldest
votes
1 Answer
1
active
oldest
votes
active
oldest
votes
active
oldest
votes
$begingroup$
Transliterations of Ukrainian names have different avatars. You can find Kravchuk polynomials, and other papers like On Krawtchouk Transforms or Krawtchouk polynomials and Krawtchouk matrices. You can find as well Kravchuk orthogonal polynomials.
As they form an orthogonal basis of polynomials (as well as many others, listed on polpak: Bernoulli, Bernstein, Chebyshev, Hermite, Laguerre, Legendre, Zernike), they are candidates for a transform. Derived moments are used in image processing, and the following paper seems to have a wide audience:
Image analysis by Krawtchouk moments, IEEE Transactions on Image Processing 2003
A new set of orthogonal moments based on the discrete classical
Krawtchouk polynomials is introduced. The Krawtchouk polynomials are
scaled to ensure numerical stability, thus creating a set of weighted
Krawtchouk polynomials. The set of proposed Krawtchouk moments is then
derived from the weighted Krawtchouk polynomials. The orthogonality of
the proposed moments ensures minimal information redundancy. No
numerical approximation is involved in deriving the moments, since the
weighted Krawtchouk polynomials are discrete. These properties make
the Krawtchouk moments well suited as pattern features in the analysis
of two-dimensional images. It is shown that the Krawtchouk moments can
be employed to extract local features of an image, unlike other
orthogonal moments, which generally capture the global features. The
computational aspects of the moments using the recursive and symmetry
properties are discussed. The theoretical framework is validated by an
experiment on image reconstruction using Krawtchouk moments and the
results are compared to that of Zernike, pseudo-Zernike, Legendre, and
Tchebyscheff moments. Krawtchouk moment invariants are constructed
using a linear combination of geometric moment invariants; an object
recognition experiment shows Krawtchouk moment invariants perform
significantly better than Hu's moment invariants in both noise-free
and noisy conditions.
Later, you can read:
Image Analysis Using Hahn Moments, IEEE Transactions on Pattern Analysis and Machine, 2007, where Hahn moments generalize Chebyshev and Krawtchouk moments:
This paper shows how Hahn moments provide a unified understanding of
the recently introduced Chebyshev and Krawtchouk moments. The two
latter moments can be obtained as particular cases of Hahn moments
with the appropriate parameter settings and this fact implies that
Hahn moments encompass all their properties. The aim of this paper is
twofold: (1) To show how Hahn moments, as a generalization of
Chebyshev and Krawtchouk moments, can be used for global and local
feature extraction and (2) to show how Hahn moments can be
incorporated into the framework of normalized convolution to analyze
local structures of irregularly sampled signals.
In Wikipedia's Discrete Fourier transform we find:
The choice of eigenvectors of the DFT matrix has become important in
recent years in order to define a discrete analogue of the fractional
Fourier transform—the DFT matrix can be taken to fractional powers by
exponentiating the eigenvalues (e.g., Rubio and Santhanam, 2005). For
the continuous Fourier transform, the natural orthogonal
eigenfunctions are the Hermite functions, so various discrete
analogues of these have been employed as the eigenvectors of the DFT,
such as the Kravchuk polynomials (Atakishiyev and Wolf, 1997). The
"best" choice of eigenvectors to define a fractional discrete Fourier
transform remains an open question, however.
$endgroup$
$begingroup$
Can we said that "Kravchouk transform" usually is a "fractional Fourier transform"?
$endgroup$
– Machupicchu
8 hours ago
$begingroup$
There are ambiguities on what people call "fractional Fourier transforms", and I am not a practitioner of the Fractional Fourier–Kravchuk transform, but I doubt there is a one-to-one correspondance
$endgroup$
– Laurent Duval
7 hours ago
1
$begingroup$
ah ok. It seems strange that they cite this obscure Kravchouk in phys.org/news/2019-07-quantum-technology.html as it does not seem to be known by many DSP people ?
$endgroup$
– Machupicchu
7 hours ago
1
$begingroup$
oh ok. So you would say it is "overstated" as important?
$endgroup$
– Machupicchu
7 hours ago
1
$begingroup$
This is another area where keeping the distinction and relationship between the discrete and continuous case is important. I like to use Legendre polynomials. I tend to think of them as "the orthogonal Taylor series", however, the discrete version in not orthogonal. You need to apply something like Gram-Schmidt (G-S) to rectify that for discrete usage. Then keeping the same N becomes important.
$endgroup$
– Cedron Dawg
7 hours ago
|
show 2 more comments
$begingroup$
Transliterations of Ukrainian names have different avatars. You can find Kravchuk polynomials, and other papers like On Krawtchouk Transforms or Krawtchouk polynomials and Krawtchouk matrices. You can find as well Kravchuk orthogonal polynomials.
As they form an orthogonal basis of polynomials (as well as many others, listed on polpak: Bernoulli, Bernstein, Chebyshev, Hermite, Laguerre, Legendre, Zernike), they are candidates for a transform. Derived moments are used in image processing, and the following paper seems to have a wide audience:
Image analysis by Krawtchouk moments, IEEE Transactions on Image Processing 2003
A new set of orthogonal moments based on the discrete classical
Krawtchouk polynomials is introduced. The Krawtchouk polynomials are
scaled to ensure numerical stability, thus creating a set of weighted
Krawtchouk polynomials. The set of proposed Krawtchouk moments is then
derived from the weighted Krawtchouk polynomials. The orthogonality of
the proposed moments ensures minimal information redundancy. No
numerical approximation is involved in deriving the moments, since the
weighted Krawtchouk polynomials are discrete. These properties make
the Krawtchouk moments well suited as pattern features in the analysis
of two-dimensional images. It is shown that the Krawtchouk moments can
be employed to extract local features of an image, unlike other
orthogonal moments, which generally capture the global features. The
computational aspects of the moments using the recursive and symmetry
properties are discussed. The theoretical framework is validated by an
experiment on image reconstruction using Krawtchouk moments and the
results are compared to that of Zernike, pseudo-Zernike, Legendre, and
Tchebyscheff moments. Krawtchouk moment invariants are constructed
using a linear combination of geometric moment invariants; an object
recognition experiment shows Krawtchouk moment invariants perform
significantly better than Hu's moment invariants in both noise-free
and noisy conditions.
Later, you can read:
Image Analysis Using Hahn Moments, IEEE Transactions on Pattern Analysis and Machine, 2007, where Hahn moments generalize Chebyshev and Krawtchouk moments:
This paper shows how Hahn moments provide a unified understanding of
the recently introduced Chebyshev and Krawtchouk moments. The two
latter moments can be obtained as particular cases of Hahn moments
with the appropriate parameter settings and this fact implies that
Hahn moments encompass all their properties. The aim of this paper is
twofold: (1) To show how Hahn moments, as a generalization of
Chebyshev and Krawtchouk moments, can be used for global and local
feature extraction and (2) to show how Hahn moments can be
incorporated into the framework of normalized convolution to analyze
local structures of irregularly sampled signals.
In Wikipedia's Discrete Fourier transform we find:
The choice of eigenvectors of the DFT matrix has become important in
recent years in order to define a discrete analogue of the fractional
Fourier transform—the DFT matrix can be taken to fractional powers by
exponentiating the eigenvalues (e.g., Rubio and Santhanam, 2005). For
the continuous Fourier transform, the natural orthogonal
eigenfunctions are the Hermite functions, so various discrete
analogues of these have been employed as the eigenvectors of the DFT,
such as the Kravchuk polynomials (Atakishiyev and Wolf, 1997). The
"best" choice of eigenvectors to define a fractional discrete Fourier
transform remains an open question, however.
$endgroup$
$begingroup$
Can we said that "Kravchouk transform" usually is a "fractional Fourier transform"?
$endgroup$
– Machupicchu
8 hours ago
$begingroup$
There are ambiguities on what people call "fractional Fourier transforms", and I am not a practitioner of the Fractional Fourier–Kravchuk transform, but I doubt there is a one-to-one correspondance
$endgroup$
– Laurent Duval
7 hours ago
1
$begingroup$
ah ok. It seems strange that they cite this obscure Kravchouk in phys.org/news/2019-07-quantum-technology.html as it does not seem to be known by many DSP people ?
$endgroup$
– Machupicchu
7 hours ago
1
$begingroup$
oh ok. So you would say it is "overstated" as important?
$endgroup$
– Machupicchu
7 hours ago
1
$begingroup$
This is another area where keeping the distinction and relationship between the discrete and continuous case is important. I like to use Legendre polynomials. I tend to think of them as "the orthogonal Taylor series", however, the discrete version in not orthogonal. You need to apply something like Gram-Schmidt (G-S) to rectify that for discrete usage. Then keeping the same N becomes important.
$endgroup$
– Cedron Dawg
7 hours ago
|
show 2 more comments
$begingroup$
Transliterations of Ukrainian names have different avatars. You can find Kravchuk polynomials, and other papers like On Krawtchouk Transforms or Krawtchouk polynomials and Krawtchouk matrices. You can find as well Kravchuk orthogonal polynomials.
As they form an orthogonal basis of polynomials (as well as many others, listed on polpak: Bernoulli, Bernstein, Chebyshev, Hermite, Laguerre, Legendre, Zernike), they are candidates for a transform. Derived moments are used in image processing, and the following paper seems to have a wide audience:
Image analysis by Krawtchouk moments, IEEE Transactions on Image Processing 2003
A new set of orthogonal moments based on the discrete classical
Krawtchouk polynomials is introduced. The Krawtchouk polynomials are
scaled to ensure numerical stability, thus creating a set of weighted
Krawtchouk polynomials. The set of proposed Krawtchouk moments is then
derived from the weighted Krawtchouk polynomials. The orthogonality of
the proposed moments ensures minimal information redundancy. No
numerical approximation is involved in deriving the moments, since the
weighted Krawtchouk polynomials are discrete. These properties make
the Krawtchouk moments well suited as pattern features in the analysis
of two-dimensional images. It is shown that the Krawtchouk moments can
be employed to extract local features of an image, unlike other
orthogonal moments, which generally capture the global features. The
computational aspects of the moments using the recursive and symmetry
properties are discussed. The theoretical framework is validated by an
experiment on image reconstruction using Krawtchouk moments and the
results are compared to that of Zernike, pseudo-Zernike, Legendre, and
Tchebyscheff moments. Krawtchouk moment invariants are constructed
using a linear combination of geometric moment invariants; an object
recognition experiment shows Krawtchouk moment invariants perform
significantly better than Hu's moment invariants in both noise-free
and noisy conditions.
Later, you can read:
Image Analysis Using Hahn Moments, IEEE Transactions on Pattern Analysis and Machine, 2007, where Hahn moments generalize Chebyshev and Krawtchouk moments:
This paper shows how Hahn moments provide a unified understanding of
the recently introduced Chebyshev and Krawtchouk moments. The two
latter moments can be obtained as particular cases of Hahn moments
with the appropriate parameter settings and this fact implies that
Hahn moments encompass all their properties. The aim of this paper is
twofold: (1) To show how Hahn moments, as a generalization of
Chebyshev and Krawtchouk moments, can be used for global and local
feature extraction and (2) to show how Hahn moments can be
incorporated into the framework of normalized convolution to analyze
local structures of irregularly sampled signals.
In Wikipedia's Discrete Fourier transform we find:
The choice of eigenvectors of the DFT matrix has become important in
recent years in order to define a discrete analogue of the fractional
Fourier transform—the DFT matrix can be taken to fractional powers by
exponentiating the eigenvalues (e.g., Rubio and Santhanam, 2005). For
the continuous Fourier transform, the natural orthogonal
eigenfunctions are the Hermite functions, so various discrete
analogues of these have been employed as the eigenvectors of the DFT,
such as the Kravchuk polynomials (Atakishiyev and Wolf, 1997). The
"best" choice of eigenvectors to define a fractional discrete Fourier
transform remains an open question, however.
$endgroup$
Transliterations of Ukrainian names have different avatars. You can find Kravchuk polynomials, and other papers like On Krawtchouk Transforms or Krawtchouk polynomials and Krawtchouk matrices. You can find as well Kravchuk orthogonal polynomials.
As they form an orthogonal basis of polynomials (as well as many others, listed on polpak: Bernoulli, Bernstein, Chebyshev, Hermite, Laguerre, Legendre, Zernike), they are candidates for a transform. Derived moments are used in image processing, and the following paper seems to have a wide audience:
Image analysis by Krawtchouk moments, IEEE Transactions on Image Processing 2003
A new set of orthogonal moments based on the discrete classical
Krawtchouk polynomials is introduced. The Krawtchouk polynomials are
scaled to ensure numerical stability, thus creating a set of weighted
Krawtchouk polynomials. The set of proposed Krawtchouk moments is then
derived from the weighted Krawtchouk polynomials. The orthogonality of
the proposed moments ensures minimal information redundancy. No
numerical approximation is involved in deriving the moments, since the
weighted Krawtchouk polynomials are discrete. These properties make
the Krawtchouk moments well suited as pattern features in the analysis
of two-dimensional images. It is shown that the Krawtchouk moments can
be employed to extract local features of an image, unlike other
orthogonal moments, which generally capture the global features. The
computational aspects of the moments using the recursive and symmetry
properties are discussed. The theoretical framework is validated by an
experiment on image reconstruction using Krawtchouk moments and the
results are compared to that of Zernike, pseudo-Zernike, Legendre, and
Tchebyscheff moments. Krawtchouk moment invariants are constructed
using a linear combination of geometric moment invariants; an object
recognition experiment shows Krawtchouk moment invariants perform
significantly better than Hu's moment invariants in both noise-free
and noisy conditions.
Later, you can read:
Image Analysis Using Hahn Moments, IEEE Transactions on Pattern Analysis and Machine, 2007, where Hahn moments generalize Chebyshev and Krawtchouk moments:
This paper shows how Hahn moments provide a unified understanding of
the recently introduced Chebyshev and Krawtchouk moments. The two
latter moments can be obtained as particular cases of Hahn moments
with the appropriate parameter settings and this fact implies that
Hahn moments encompass all their properties. The aim of this paper is
twofold: (1) To show how Hahn moments, as a generalization of
Chebyshev and Krawtchouk moments, can be used for global and local
feature extraction and (2) to show how Hahn moments can be
incorporated into the framework of normalized convolution to analyze
local structures of irregularly sampled signals.
In Wikipedia's Discrete Fourier transform we find:
The choice of eigenvectors of the DFT matrix has become important in
recent years in order to define a discrete analogue of the fractional
Fourier transform—the DFT matrix can be taken to fractional powers by
exponentiating the eigenvalues (e.g., Rubio and Santhanam, 2005). For
the continuous Fourier transform, the natural orthogonal
eigenfunctions are the Hermite functions, so various discrete
analogues of these have been employed as the eigenvectors of the DFT,
such as the Kravchuk polynomials (Atakishiyev and Wolf, 1997). The
"best" choice of eigenvectors to define a fractional discrete Fourier
transform remains an open question, however.
edited 7 hours ago
answered 8 hours ago


Laurent DuvalLaurent Duval
17.7k3 gold badges21 silver badges67 bronze badges
17.7k3 gold badges21 silver badges67 bronze badges
$begingroup$
Can we said that "Kravchouk transform" usually is a "fractional Fourier transform"?
$endgroup$
– Machupicchu
8 hours ago
$begingroup$
There are ambiguities on what people call "fractional Fourier transforms", and I am not a practitioner of the Fractional Fourier–Kravchuk transform, but I doubt there is a one-to-one correspondance
$endgroup$
– Laurent Duval
7 hours ago
1
$begingroup$
ah ok. It seems strange that they cite this obscure Kravchouk in phys.org/news/2019-07-quantum-technology.html as it does not seem to be known by many DSP people ?
$endgroup$
– Machupicchu
7 hours ago
1
$begingroup$
oh ok. So you would say it is "overstated" as important?
$endgroup$
– Machupicchu
7 hours ago
1
$begingroup$
This is another area where keeping the distinction and relationship between the discrete and continuous case is important. I like to use Legendre polynomials. I tend to think of them as "the orthogonal Taylor series", however, the discrete version in not orthogonal. You need to apply something like Gram-Schmidt (G-S) to rectify that for discrete usage. Then keeping the same N becomes important.
$endgroup$
– Cedron Dawg
7 hours ago
|
show 2 more comments
$begingroup$
Can we said that "Kravchouk transform" usually is a "fractional Fourier transform"?
$endgroup$
– Machupicchu
8 hours ago
$begingroup$
There are ambiguities on what people call "fractional Fourier transforms", and I am not a practitioner of the Fractional Fourier–Kravchuk transform, but I doubt there is a one-to-one correspondance
$endgroup$
– Laurent Duval
7 hours ago
1
$begingroup$
ah ok. It seems strange that they cite this obscure Kravchouk in phys.org/news/2019-07-quantum-technology.html as it does not seem to be known by many DSP people ?
$endgroup$
– Machupicchu
7 hours ago
1
$begingroup$
oh ok. So you would say it is "overstated" as important?
$endgroup$
– Machupicchu
7 hours ago
1
$begingroup$
This is another area where keeping the distinction and relationship between the discrete and continuous case is important. I like to use Legendre polynomials. I tend to think of them as "the orthogonal Taylor series", however, the discrete version in not orthogonal. You need to apply something like Gram-Schmidt (G-S) to rectify that for discrete usage. Then keeping the same N becomes important.
$endgroup$
– Cedron Dawg
7 hours ago
$begingroup$
Can we said that "Kravchouk transform" usually is a "fractional Fourier transform"?
$endgroup$
– Machupicchu
8 hours ago
$begingroup$
Can we said that "Kravchouk transform" usually is a "fractional Fourier transform"?
$endgroup$
– Machupicchu
8 hours ago
$begingroup$
There are ambiguities on what people call "fractional Fourier transforms", and I am not a practitioner of the Fractional Fourier–Kravchuk transform, but I doubt there is a one-to-one correspondance
$endgroup$
– Laurent Duval
7 hours ago
$begingroup$
There are ambiguities on what people call "fractional Fourier transforms", and I am not a practitioner of the Fractional Fourier–Kravchuk transform, but I doubt there is a one-to-one correspondance
$endgroup$
– Laurent Duval
7 hours ago
1
1
$begingroup$
ah ok. It seems strange that they cite this obscure Kravchouk in phys.org/news/2019-07-quantum-technology.html as it does not seem to be known by many DSP people ?
$endgroup$
– Machupicchu
7 hours ago
$begingroup$
ah ok. It seems strange that they cite this obscure Kravchouk in phys.org/news/2019-07-quantum-technology.html as it does not seem to be known by many DSP people ?
$endgroup$
– Machupicchu
7 hours ago
1
1
$begingroup$
oh ok. So you would say it is "overstated" as important?
$endgroup$
– Machupicchu
7 hours ago
$begingroup$
oh ok. So you would say it is "overstated" as important?
$endgroup$
– Machupicchu
7 hours ago
1
1
$begingroup$
This is another area where keeping the distinction and relationship between the discrete and continuous case is important. I like to use Legendre polynomials. I tend to think of them as "the orthogonal Taylor series", however, the discrete version in not orthogonal. You need to apply something like Gram-Schmidt (G-S) to rectify that for discrete usage. Then keeping the same N becomes important.
$endgroup$
– Cedron Dawg
7 hours ago
$begingroup$
This is another area where keeping the distinction and relationship between the discrete and continuous case is important. I like to use Legendre polynomials. I tend to think of them as "the orthogonal Taylor series", however, the discrete version in not orthogonal. You need to apply something like Gram-Schmidt (G-S) to rectify that for discrete usage. Then keeping the same N becomes important.
$endgroup$
– Cedron Dawg
7 hours ago
|
show 2 more comments
Thanks for contributing an answer to Signal Processing Stack Exchange!
- Please be sure to answer the question. Provide details and share your research!
But avoid …
- Asking for help, clarification, or responding to other answers.
- Making statements based on opinion; back them up with references or personal experience.
Use MathJax to format equations. MathJax reference.
To learn more, see our tips on writing great answers.
Sign up or log in
StackExchange.ready(function ()
StackExchange.helpers.onClickDraftSave('#login-link');
);
Sign up using Google
Sign up using Facebook
Sign up using Email and Password
Post as a guest
Required, but never shown
StackExchange.ready(
function ()
StackExchange.openid.initPostLogin('.new-post-login', 'https%3a%2f%2fdsp.stackexchange.com%2fquestions%2f59657%2fwhat-is-a-kravchuk-transform-and-how-is-it-related-to-fourier-transforms%23new-answer', 'question_page');
);
Post as a guest
Required, but never shown
Sign up or log in
StackExchange.ready(function ()
StackExchange.helpers.onClickDraftSave('#login-link');
);
Sign up using Google
Sign up using Facebook
Sign up using Email and Password
Post as a guest
Required, but never shown
Sign up or log in
StackExchange.ready(function ()
StackExchange.helpers.onClickDraftSave('#login-link');
);
Sign up using Google
Sign up using Facebook
Sign up using Email and Password
Post as a guest
Required, but never shown
Sign up or log in
StackExchange.ready(function ()
StackExchange.helpers.onClickDraftSave('#login-link');
);
Sign up using Google
Sign up using Facebook
Sign up using Email and Password
Sign up using Google
Sign up using Facebook
Sign up using Email and Password
Post as a guest
Required, but never shown
Required, but never shown
Required, but never shown
Required, but never shown
Required, but never shown
Required, but never shown
Required, but never shown
Required, but never shown
Required, but never shown
LldiQjCaDQIMREE,0a69NyTjHQoN uTNmCmYRCZLdSfXeINk