Multiple tests with effects all in same direction but only few significantWeighting a control groupAnova repeated measures is significant, but all the multiple comparisons with Bonferroni correction are not?If I perform multiple unpaired t-tests on data, then break it down by gender, do I need to do additional multiple correction?When to create a control group with paired T testComparing Functional Connectivity as measured by fMRIWhat statistical test do I use to know the difference between treatment and control changes across a group? How many subjects would I need?Sample size analysis for pearson's R - corrected for multiple comparisonDivide patients into 2 groupsHypothesis testing control vs treatment when the outcome tends to vary over timeLow sample size analysis with all effects in same direction
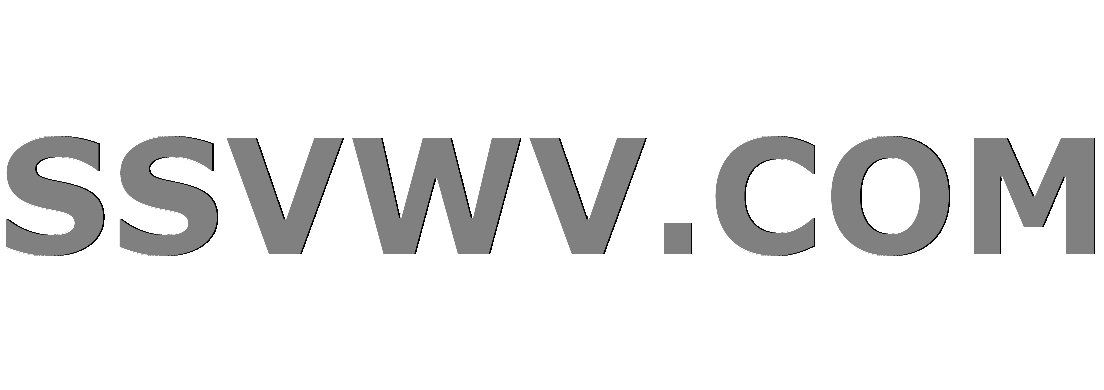
Multi tool use
Why would Dementors torture a Death Eater if they are loyal to Voldemort?
Processes in a session in an interactive shell vs in a script
Rear derailleur got caught in the spokes, what could be a root cause
Basic calculations in PGF/TikZ for loop
iMac 2019: Can I mix the old modules with the new ones when upgrading RAM?
Word ending in "-ine" for rat-like
Understanding the as-if rule, "the program was executed as written"
How do I present a future free of gender stereotypes without being jarring or overpowering the narrative?
Disk usage buggy: 10G missing on Linux home partition on SSD
Could you fall off a planet if it was being accelerated by engines?
Installed software from source, how to say yum not to install it from package?
Why did the Apple IIe make a hideous noise if you inserted the disk upside down?
Chandra exiles a card, I play it, it gets exiled again
Is it advisable to inform the CEO about his brother accessing his office?
How to stop QGIS from looking for the wrong PostgreSQL host address in an existing workproject?
Drawing a sigmoid function and its derivative in tikz
Why are symbols not written in words?
Why will we fail creating a self sustaining off world colony?
What prevents a US state from colonizing a smaller state?
What verb goes with "coup"?
Why doesn't SpaceX land boosters in Africa?
Calculus, water poured into a cone: Why is the derivative non-linear?
Robots in a spaceship
Are you required to spend hit dice to take a short rest?
Multiple tests with effects all in same direction but only few significant
Weighting a control groupAnova repeated measures is significant, but all the multiple comparisons with Bonferroni correction are not?If I perform multiple unpaired t-tests on data, then break it down by gender, do I need to do additional multiple correction?When to create a control group with paired T testComparing Functional Connectivity as measured by fMRIWhat statistical test do I use to know the difference between treatment and control changes across a group? How many subjects would I need?Sample size analysis for pearson's R - corrected for multiple comparisonDivide patients into 2 groupsHypothesis testing control vs treatment when the outcome tends to vary over timeLow sample size analysis with all effects in same direction
$begingroup$
I have tests done looking at the brain activity in 12 different regions of the brain. It is a between subject design where there is a treatment group (N = 8) and control group (N = 14). Each participant had these scans done on the 12 different brain regions. Every effect for all 12 brain regions showed that the treatment group had higher brain activity than the control group. However, the problem is because of the low sample size only 2 of these effects were significant with the others p values somewhere between .05 and .20. From this is there any statistical analysis I can do to be able to say that overall the brain activity was higher in the treatment group compared to the control? I tried taking an average activity among the 12 brain regions for each individual and analyzing that but the sample size was still N = 8 and N = 14 so it wasnt significant. Would it be possible to combine all of the data together and do a t test on that making it N = 8*12 = 96 for the treatment group and N = 14 * 12 = 168 for the control?
hypothesis-testing statistical-significance clustering t-test
$endgroup$
add a comment |
$begingroup$
I have tests done looking at the brain activity in 12 different regions of the brain. It is a between subject design where there is a treatment group (N = 8) and control group (N = 14). Each participant had these scans done on the 12 different brain regions. Every effect for all 12 brain regions showed that the treatment group had higher brain activity than the control group. However, the problem is because of the low sample size only 2 of these effects were significant with the others p values somewhere between .05 and .20. From this is there any statistical analysis I can do to be able to say that overall the brain activity was higher in the treatment group compared to the control? I tried taking an average activity among the 12 brain regions for each individual and analyzing that but the sample size was still N = 8 and N = 14 so it wasnt significant. Would it be possible to combine all of the data together and do a t test on that making it N = 8*12 = 96 for the treatment group and N = 14 * 12 = 168 for the control?
hypothesis-testing statistical-significance clustering t-test
$endgroup$
add a comment |
$begingroup$
I have tests done looking at the brain activity in 12 different regions of the brain. It is a between subject design where there is a treatment group (N = 8) and control group (N = 14). Each participant had these scans done on the 12 different brain regions. Every effect for all 12 brain regions showed that the treatment group had higher brain activity than the control group. However, the problem is because of the low sample size only 2 of these effects were significant with the others p values somewhere between .05 and .20. From this is there any statistical analysis I can do to be able to say that overall the brain activity was higher in the treatment group compared to the control? I tried taking an average activity among the 12 brain regions for each individual and analyzing that but the sample size was still N = 8 and N = 14 so it wasnt significant. Would it be possible to combine all of the data together and do a t test on that making it N = 8*12 = 96 for the treatment group and N = 14 * 12 = 168 for the control?
hypothesis-testing statistical-significance clustering t-test
$endgroup$
I have tests done looking at the brain activity in 12 different regions of the brain. It is a between subject design where there is a treatment group (N = 8) and control group (N = 14). Each participant had these scans done on the 12 different brain regions. Every effect for all 12 brain regions showed that the treatment group had higher brain activity than the control group. However, the problem is because of the low sample size only 2 of these effects were significant with the others p values somewhere between .05 and .20. From this is there any statistical analysis I can do to be able to say that overall the brain activity was higher in the treatment group compared to the control? I tried taking an average activity among the 12 brain regions for each individual and analyzing that but the sample size was still N = 8 and N = 14 so it wasnt significant. Would it be possible to combine all of the data together and do a t test on that making it N = 8*12 = 96 for the treatment group and N = 14 * 12 = 168 for the control?
hypothesis-testing statistical-significance clustering t-test
hypothesis-testing statistical-significance clustering t-test
asked 8 hours ago
RyanRyan
345 bronze badges
345 bronze badges
add a comment |
add a comment |
1 Answer
1
active
oldest
votes
$begingroup$
There seem to be at least two important difficulties:
(1) Small sample sizes. Given that you could afford to look at 22 subjects, a (slightly) more efficient design would have been to have 11 in each group. But it may just be easier to enroll Control subjects than Treatment subjects.
(2) Lack of independence. It is not clear whether results from various regions of the brain are independent. If the tests at 12 regions were independent, then having all 12 go in the same direction would be convincing. Without independence, the exact suggestion in your last sentence seems off the table.
Some suggestions:
Although the 5% significant level may be crucial for journal editors,
that is an arbitrary criterion. It may be worthwhile doing further investigations when P-values are around 10%.
You don't say anything about looking at correlations of results between brain regions. It may be useful to do so. If results in some brain regions
might, in effect, be used to predict results in other regions, then (a) that may be important information in itself, or (b) maybe focusing on regions that provide useful independent information will permit looking at fewer regions and, over the long run, make larger sample sizes feasible.
You have two categories of subjects, Treatment and Control. You might try
discriminant analysis using all 12 regions to see how effectively your data
separate the two groups. Also, you might try some sort of discriminant or cluster analysis
to see if the data identify known patient 'groups' or suggest unexpected ones in 12-dimensions.
Maybe someone else on this site could offer an opinion whether 22 subjects
is likely to be enough to make such approaches worthwhile.
Notes: Perhaps, the best known example of discriminant analysis is
Fisher's introductory one.
For 50 specimens of each of three varieties of iris flowers, four measurements were made: sepal and petal lengths and widths. His analysis showed that
it is possible to classify almost all 150 specimens as to variety based only on these measurements.
Can you classify your subjects as to Treatment and Control using your 12 measurements? Distinct classification is more difficult than barely detecting a difference in group means. Also, are there obvious sub-categories within Treatment and/or Control groups that might be worthwhile looking at?
By contrast, cluster analysis seeks out distinct groups (perhaps not previously anticipated) based on data.
$endgroup$
add a comment |
Your Answer
StackExchange.ready(function()
var channelOptions =
tags: "".split(" "),
id: "65"
;
initTagRenderer("".split(" "), "".split(" "), channelOptions);
StackExchange.using("externalEditor", function()
// Have to fire editor after snippets, if snippets enabled
if (StackExchange.settings.snippets.snippetsEnabled)
StackExchange.using("snippets", function()
createEditor();
);
else
createEditor();
);
function createEditor()
StackExchange.prepareEditor(
heartbeatType: 'answer',
autoActivateHeartbeat: false,
convertImagesToLinks: false,
noModals: true,
showLowRepImageUploadWarning: true,
reputationToPostImages: null,
bindNavPrevention: true,
postfix: "",
imageUploader:
brandingHtml: "Powered by u003ca class="icon-imgur-white" href="https://imgur.com/"u003eu003c/au003e",
contentPolicyHtml: "User contributions licensed under u003ca href="https://creativecommons.org/licenses/by-sa/3.0/"u003ecc by-sa 3.0 with attribution requiredu003c/au003e u003ca href="https://stackoverflow.com/legal/content-policy"u003e(content policy)u003c/au003e",
allowUrls: true
,
onDemand: true,
discardSelector: ".discard-answer"
,immediatelyShowMarkdownHelp:true
);
);
Sign up or log in
StackExchange.ready(function ()
StackExchange.helpers.onClickDraftSave('#login-link');
);
Sign up using Google
Sign up using Facebook
Sign up using Email and Password
Post as a guest
Required, but never shown
StackExchange.ready(
function ()
StackExchange.openid.initPostLogin('.new-post-login', 'https%3a%2f%2fstats.stackexchange.com%2fquestions%2f415323%2fmultiple-tests-with-effects-all-in-same-direction-but-only-few-significant%23new-answer', 'question_page');
);
Post as a guest
Required, but never shown
1 Answer
1
active
oldest
votes
1 Answer
1
active
oldest
votes
active
oldest
votes
active
oldest
votes
$begingroup$
There seem to be at least two important difficulties:
(1) Small sample sizes. Given that you could afford to look at 22 subjects, a (slightly) more efficient design would have been to have 11 in each group. But it may just be easier to enroll Control subjects than Treatment subjects.
(2) Lack of independence. It is not clear whether results from various regions of the brain are independent. If the tests at 12 regions were independent, then having all 12 go in the same direction would be convincing. Without independence, the exact suggestion in your last sentence seems off the table.
Some suggestions:
Although the 5% significant level may be crucial for journal editors,
that is an arbitrary criterion. It may be worthwhile doing further investigations when P-values are around 10%.
You don't say anything about looking at correlations of results between brain regions. It may be useful to do so. If results in some brain regions
might, in effect, be used to predict results in other regions, then (a) that may be important information in itself, or (b) maybe focusing on regions that provide useful independent information will permit looking at fewer regions and, over the long run, make larger sample sizes feasible.
You have two categories of subjects, Treatment and Control. You might try
discriminant analysis using all 12 regions to see how effectively your data
separate the two groups. Also, you might try some sort of discriminant or cluster analysis
to see if the data identify known patient 'groups' or suggest unexpected ones in 12-dimensions.
Maybe someone else on this site could offer an opinion whether 22 subjects
is likely to be enough to make such approaches worthwhile.
Notes: Perhaps, the best known example of discriminant analysis is
Fisher's introductory one.
For 50 specimens of each of three varieties of iris flowers, four measurements were made: sepal and petal lengths and widths. His analysis showed that
it is possible to classify almost all 150 specimens as to variety based only on these measurements.
Can you classify your subjects as to Treatment and Control using your 12 measurements? Distinct classification is more difficult than barely detecting a difference in group means. Also, are there obvious sub-categories within Treatment and/or Control groups that might be worthwhile looking at?
By contrast, cluster analysis seeks out distinct groups (perhaps not previously anticipated) based on data.
$endgroup$
add a comment |
$begingroup$
There seem to be at least two important difficulties:
(1) Small sample sizes. Given that you could afford to look at 22 subjects, a (slightly) more efficient design would have been to have 11 in each group. But it may just be easier to enroll Control subjects than Treatment subjects.
(2) Lack of independence. It is not clear whether results from various regions of the brain are independent. If the tests at 12 regions were independent, then having all 12 go in the same direction would be convincing. Without independence, the exact suggestion in your last sentence seems off the table.
Some suggestions:
Although the 5% significant level may be crucial for journal editors,
that is an arbitrary criterion. It may be worthwhile doing further investigations when P-values are around 10%.
You don't say anything about looking at correlations of results between brain regions. It may be useful to do so. If results in some brain regions
might, in effect, be used to predict results in other regions, then (a) that may be important information in itself, or (b) maybe focusing on regions that provide useful independent information will permit looking at fewer regions and, over the long run, make larger sample sizes feasible.
You have two categories of subjects, Treatment and Control. You might try
discriminant analysis using all 12 regions to see how effectively your data
separate the two groups. Also, you might try some sort of discriminant or cluster analysis
to see if the data identify known patient 'groups' or suggest unexpected ones in 12-dimensions.
Maybe someone else on this site could offer an opinion whether 22 subjects
is likely to be enough to make such approaches worthwhile.
Notes: Perhaps, the best known example of discriminant analysis is
Fisher's introductory one.
For 50 specimens of each of three varieties of iris flowers, four measurements were made: sepal and petal lengths and widths. His analysis showed that
it is possible to classify almost all 150 specimens as to variety based only on these measurements.
Can you classify your subjects as to Treatment and Control using your 12 measurements? Distinct classification is more difficult than barely detecting a difference in group means. Also, are there obvious sub-categories within Treatment and/or Control groups that might be worthwhile looking at?
By contrast, cluster analysis seeks out distinct groups (perhaps not previously anticipated) based on data.
$endgroup$
add a comment |
$begingroup$
There seem to be at least two important difficulties:
(1) Small sample sizes. Given that you could afford to look at 22 subjects, a (slightly) more efficient design would have been to have 11 in each group. But it may just be easier to enroll Control subjects than Treatment subjects.
(2) Lack of independence. It is not clear whether results from various regions of the brain are independent. If the tests at 12 regions were independent, then having all 12 go in the same direction would be convincing. Without independence, the exact suggestion in your last sentence seems off the table.
Some suggestions:
Although the 5% significant level may be crucial for journal editors,
that is an arbitrary criterion. It may be worthwhile doing further investigations when P-values are around 10%.
You don't say anything about looking at correlations of results between brain regions. It may be useful to do so. If results in some brain regions
might, in effect, be used to predict results in other regions, then (a) that may be important information in itself, or (b) maybe focusing on regions that provide useful independent information will permit looking at fewer regions and, over the long run, make larger sample sizes feasible.
You have two categories of subjects, Treatment and Control. You might try
discriminant analysis using all 12 regions to see how effectively your data
separate the two groups. Also, you might try some sort of discriminant or cluster analysis
to see if the data identify known patient 'groups' or suggest unexpected ones in 12-dimensions.
Maybe someone else on this site could offer an opinion whether 22 subjects
is likely to be enough to make such approaches worthwhile.
Notes: Perhaps, the best known example of discriminant analysis is
Fisher's introductory one.
For 50 specimens of each of three varieties of iris flowers, four measurements were made: sepal and petal lengths and widths. His analysis showed that
it is possible to classify almost all 150 specimens as to variety based only on these measurements.
Can you classify your subjects as to Treatment and Control using your 12 measurements? Distinct classification is more difficult than barely detecting a difference in group means. Also, are there obvious sub-categories within Treatment and/or Control groups that might be worthwhile looking at?
By contrast, cluster analysis seeks out distinct groups (perhaps not previously anticipated) based on data.
$endgroup$
There seem to be at least two important difficulties:
(1) Small sample sizes. Given that you could afford to look at 22 subjects, a (slightly) more efficient design would have been to have 11 in each group. But it may just be easier to enroll Control subjects than Treatment subjects.
(2) Lack of independence. It is not clear whether results from various regions of the brain are independent. If the tests at 12 regions were independent, then having all 12 go in the same direction would be convincing. Without independence, the exact suggestion in your last sentence seems off the table.
Some suggestions:
Although the 5% significant level may be crucial for journal editors,
that is an arbitrary criterion. It may be worthwhile doing further investigations when P-values are around 10%.
You don't say anything about looking at correlations of results between brain regions. It may be useful to do so. If results in some brain regions
might, in effect, be used to predict results in other regions, then (a) that may be important information in itself, or (b) maybe focusing on regions that provide useful independent information will permit looking at fewer regions and, over the long run, make larger sample sizes feasible.
You have two categories of subjects, Treatment and Control. You might try
discriminant analysis using all 12 regions to see how effectively your data
separate the two groups. Also, you might try some sort of discriminant or cluster analysis
to see if the data identify known patient 'groups' or suggest unexpected ones in 12-dimensions.
Maybe someone else on this site could offer an opinion whether 22 subjects
is likely to be enough to make such approaches worthwhile.
Notes: Perhaps, the best known example of discriminant analysis is
Fisher's introductory one.
For 50 specimens of each of three varieties of iris flowers, four measurements were made: sepal and petal lengths and widths. His analysis showed that
it is possible to classify almost all 150 specimens as to variety based only on these measurements.
Can you classify your subjects as to Treatment and Control using your 12 measurements? Distinct classification is more difficult than barely detecting a difference in group means. Also, are there obvious sub-categories within Treatment and/or Control groups that might be worthwhile looking at?
By contrast, cluster analysis seeks out distinct groups (perhaps not previously anticipated) based on data.
answered 7 hours ago
BruceETBruceET
9,8621 gold badge8 silver badges24 bronze badges
9,8621 gold badge8 silver badges24 bronze badges
add a comment |
add a comment |
Thanks for contributing an answer to Cross Validated!
- Please be sure to answer the question. Provide details and share your research!
But avoid …
- Asking for help, clarification, or responding to other answers.
- Making statements based on opinion; back them up with references or personal experience.
Use MathJax to format equations. MathJax reference.
To learn more, see our tips on writing great answers.
Sign up or log in
StackExchange.ready(function ()
StackExchange.helpers.onClickDraftSave('#login-link');
);
Sign up using Google
Sign up using Facebook
Sign up using Email and Password
Post as a guest
Required, but never shown
StackExchange.ready(
function ()
StackExchange.openid.initPostLogin('.new-post-login', 'https%3a%2f%2fstats.stackexchange.com%2fquestions%2f415323%2fmultiple-tests-with-effects-all-in-same-direction-but-only-few-significant%23new-answer', 'question_page');
);
Post as a guest
Required, but never shown
Sign up or log in
StackExchange.ready(function ()
StackExchange.helpers.onClickDraftSave('#login-link');
);
Sign up using Google
Sign up using Facebook
Sign up using Email and Password
Post as a guest
Required, but never shown
Sign up or log in
StackExchange.ready(function ()
StackExchange.helpers.onClickDraftSave('#login-link');
);
Sign up using Google
Sign up using Facebook
Sign up using Email and Password
Post as a guest
Required, but never shown
Sign up or log in
StackExchange.ready(function ()
StackExchange.helpers.onClickDraftSave('#login-link');
);
Sign up using Google
Sign up using Facebook
Sign up using Email and Password
Sign up using Google
Sign up using Facebook
Sign up using Email and Password
Post as a guest
Required, but never shown
Required, but never shown
Required, but never shown
Required, but never shown
Required, but never shown
Required, but never shown
Required, but never shown
Required, but never shown
Required, but never shown
Gpmi9Q ns,k5Rnaka,i,OW3J