Is This Constraint Convex?Reference for “expectation preserves convexity”Static stochastic knapsack problem: unbounded versionHow to determine the convexity of my problem and categorize it?Convex vs Strictly Quasiconvex Functions in OptimizationConvexity of Variance MinimizationCan an integer optimization problem be convex?How to linearize a constraint with maxHow To Linearize $X = maxx_1,x_2$Examples of problems with non-convex constraint functions but convex feasible region
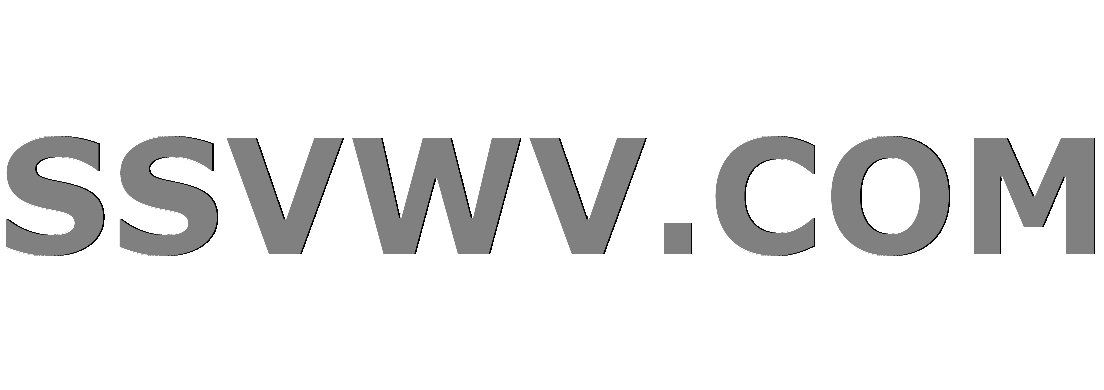
Multi tool use
How to explain to traditional people why they should upgrade their old Windows XP device?
Why is there potato in meatballs?
Conservation of momentum in photon-atom collision
A new type of builder pattern
Automatically verify passwords for classroom exercise?
How to tell my mentor to be careful when discussing my work in progress around people I do not trust?
What are the minimum element requirements for a star?
Ethics: Is it ethical for a professor to conduct research using a student's ideas without giving them credit?
How does the credit rating of a country affect its populace?
Grammar explanation for ~よし
How to capitalize letter (using uppercase) that has been assigned to let (or to def)?
Car imitates dead battery but comes back to life ~30 minutes later and lets me start it
What was the motive for inventing Gröbner bases?
Does toddler keep hands around private parts?
Is it possible to change paper title after send back from reviewers for revising?
Twelve Labours #08 - Dark Horse Bookmakers
What Are The Semantics Of Wav-File Sample Values?
Isn't any conversation with the US president quid-pro-quo?
What does Yoda's species eat?
Is every conformal manifold equivalent to a flat one with cone singularities?
Need help reading piano sheet music
RPMs too high on freeway?
Speed up animation
My PhD defense is next week and I am having negative thoughts about my work and knowledge. Any advice on how to tackle this?
Is This Constraint Convex?
Reference for “expectation preserves convexity”Static stochastic knapsack problem: unbounded versionHow to determine the convexity of my problem and categorize it?Convex vs Strictly Quasiconvex Functions in OptimizationConvexity of Variance MinimizationCan an integer optimization problem be convex?How to linearize a constraint with maxHow To Linearize $X = maxx_1,x_2$Examples of problems with non-convex constraint functions but convex feasible region
$begingroup$
I have a constraint that I believe to be convex and not affine which I think means that I can implement a relaxation. I will first define the full constraint, and then build up my (informal) reasoning as to why I think it's convex. Hopefully the holes in my thinking can be pointed out and corrected.
$$ X_t = frac Y_t-1^2 Y_t-1^2 + a^2Z_t-1, quad t=1,2,dots,T tag1 $$
$$ X_t,Y_t,Z_t ge 0, $$
$$ X_0, Y_0, Z_0, alpha gt 0 $$
Argument 1: A quadratic polynomial is convex, so if the constraint was simply $ X_t = Y_t-1^2$ then the constraint would be convex.
Argument 2: By a similar extension, $ X_t = Y_t-1^2 + alpha^2 $ would be convex.
Argument 3: The ratio of two convex functions, call it $F_t-1$, should also be convex.
Argument 4: If $F_t-1$ is convex, then multiplying $F_t-1$ by a continuous linear variable would not impose any non-convexity issues.
Conclusion: The original constraint is convex but not affine and as such we can apply a relaxation to change the problem into:
$$ X_t le frac Y_t-1^2 Y_t-1^2 + a^2Z_t-1, quad t=1,2,dots,T tag1 $$
constraint convexity nonconvex-programming lp-relaxation convexity-propagation
$endgroup$
add a comment
|
$begingroup$
I have a constraint that I believe to be convex and not affine which I think means that I can implement a relaxation. I will first define the full constraint, and then build up my (informal) reasoning as to why I think it's convex. Hopefully the holes in my thinking can be pointed out and corrected.
$$ X_t = frac Y_t-1^2 Y_t-1^2 + a^2Z_t-1, quad t=1,2,dots,T tag1 $$
$$ X_t,Y_t,Z_t ge 0, $$
$$ X_0, Y_0, Z_0, alpha gt 0 $$
Argument 1: A quadratic polynomial is convex, so if the constraint was simply $ X_t = Y_t-1^2$ then the constraint would be convex.
Argument 2: By a similar extension, $ X_t = Y_t-1^2 + alpha^2 $ would be convex.
Argument 3: The ratio of two convex functions, call it $F_t-1$, should also be convex.
Argument 4: If $F_t-1$ is convex, then multiplying $F_t-1$ by a continuous linear variable would not impose any non-convexity issues.
Conclusion: The original constraint is convex but not affine and as such we can apply a relaxation to change the problem into:
$$ X_t le frac Y_t-1^2 Y_t-1^2 + a^2Z_t-1, quad t=1,2,dots,T tag1 $$
constraint convexity nonconvex-programming lp-relaxation convexity-propagation
$endgroup$
$begingroup$
Even though your argument is not correct, there is still a possibility to obtain a convex formulation. You could substitute all your $X_t$ variables using the equality constraints that you showed us. If you are (really) lucky, it might be that your problem is convex after the substitutions.
$endgroup$
– Kevin Dalmeijer
Oct 16 at 3:20
add a comment
|
$begingroup$
I have a constraint that I believe to be convex and not affine which I think means that I can implement a relaxation. I will first define the full constraint, and then build up my (informal) reasoning as to why I think it's convex. Hopefully the holes in my thinking can be pointed out and corrected.
$$ X_t = frac Y_t-1^2 Y_t-1^2 + a^2Z_t-1, quad t=1,2,dots,T tag1 $$
$$ X_t,Y_t,Z_t ge 0, $$
$$ X_0, Y_0, Z_0, alpha gt 0 $$
Argument 1: A quadratic polynomial is convex, so if the constraint was simply $ X_t = Y_t-1^2$ then the constraint would be convex.
Argument 2: By a similar extension, $ X_t = Y_t-1^2 + alpha^2 $ would be convex.
Argument 3: The ratio of two convex functions, call it $F_t-1$, should also be convex.
Argument 4: If $F_t-1$ is convex, then multiplying $F_t-1$ by a continuous linear variable would not impose any non-convexity issues.
Conclusion: The original constraint is convex but not affine and as such we can apply a relaxation to change the problem into:
$$ X_t le frac Y_t-1^2 Y_t-1^2 + a^2Z_t-1, quad t=1,2,dots,T tag1 $$
constraint convexity nonconvex-programming lp-relaxation convexity-propagation
$endgroup$
I have a constraint that I believe to be convex and not affine which I think means that I can implement a relaxation. I will first define the full constraint, and then build up my (informal) reasoning as to why I think it's convex. Hopefully the holes in my thinking can be pointed out and corrected.
$$ X_t = frac Y_t-1^2 Y_t-1^2 + a^2Z_t-1, quad t=1,2,dots,T tag1 $$
$$ X_t,Y_t,Z_t ge 0, $$
$$ X_0, Y_0, Z_0, alpha gt 0 $$
Argument 1: A quadratic polynomial is convex, so if the constraint was simply $ X_t = Y_t-1^2$ then the constraint would be convex.
Argument 2: By a similar extension, $ X_t = Y_t-1^2 + alpha^2 $ would be convex.
Argument 3: The ratio of two convex functions, call it $F_t-1$, should also be convex.
Argument 4: If $F_t-1$ is convex, then multiplying $F_t-1$ by a continuous linear variable would not impose any non-convexity issues.
Conclusion: The original constraint is convex but not affine and as such we can apply a relaxation to change the problem into:
$$ X_t le frac Y_t-1^2 Y_t-1^2 + a^2Z_t-1, quad t=1,2,dots,T tag1 $$
constraint convexity nonconvex-programming lp-relaxation convexity-propagation
constraint convexity nonconvex-programming lp-relaxation convexity-propagation
edited Oct 17 at 15:45


Mark L. Stone
4,7151 gold badge10 silver badges35 bronze badges
4,7151 gold badge10 silver badges35 bronze badges
asked Oct 15 at 15:54
D.GrayD.Gray
1,70514 bronze badges
1,70514 bronze badges
$begingroup$
Even though your argument is not correct, there is still a possibility to obtain a convex formulation. You could substitute all your $X_t$ variables using the equality constraints that you showed us. If you are (really) lucky, it might be that your problem is convex after the substitutions.
$endgroup$
– Kevin Dalmeijer
Oct 16 at 3:20
add a comment
|
$begingroup$
Even though your argument is not correct, there is still a possibility to obtain a convex formulation. You could substitute all your $X_t$ variables using the equality constraints that you showed us. If you are (really) lucky, it might be that your problem is convex after the substitutions.
$endgroup$
– Kevin Dalmeijer
Oct 16 at 3:20
$begingroup$
Even though your argument is not correct, there is still a possibility to obtain a convex formulation. You could substitute all your $X_t$ variables using the equality constraints that you showed us. If you are (really) lucky, it might be that your problem is convex after the substitutions.
$endgroup$
– Kevin Dalmeijer
Oct 16 at 3:20
$begingroup$
Even though your argument is not correct, there is still a possibility to obtain a convex formulation. You could substitute all your $X_t$ variables using the equality constraints that you showed us. If you are (really) lucky, it might be that your problem is convex after the substitutions.
$endgroup$
– Kevin Dalmeijer
Oct 16 at 3:20
add a comment
|
2 Answers
2
active
oldest
votes
$begingroup$
Arguments 3 and 4 are incorrect. The Right-Hand Side (RHS) is not convex. Even if it were, setting a nonlinear equality with either side non-affine is non-convex. As the final coup de grace, even if the RHS were convex, an inequality, affine expression $le$ convex RHS, is going the wrong direction to be convex.
I suggest you study sections 2.3 and especially 3.2, both named "Operations that preserve convexity" of Convex Optimization, by Boyd and Vandenberghe which is freely available at the linked website of one of the authors.
$endgroup$
3
$begingroup$
Thank you for the resource! Always appreciate your guidance on the forums.
$endgroup$
– D.Gray
Oct 15 at 16:57
$begingroup$
Boyd also recommends (jokingly?) to work on this problem in two ways, simultaneously: While you try to prove convexity on paper, you run a program that samples two random points, and checks the convexity of the function at their midpoint, potentially providing a counter-example.
$endgroup$
– Robert Schwarz
Oct 16 at 8:41
add a comment
|
$begingroup$
Counterexamples to your arguments:
Argument 1:
Only affine equality constraints are convex, $x = y^2$ is not convex.
Argument 3:
Take $f(x) = x^4$ and $g(x) = x$. Both are convex, but the ratio $h(x) = fracx^4x $ is not.
Argument 4:
Let $f(x) = x$, and $y in mathbbR$. $f$ is convex, but $g(x, y) = yf(x) = xy$ is not.
$endgroup$
add a comment
|
Your Answer
StackExchange.ready(function()
var channelOptions =
tags: "".split(" "),
id: "700"
;
initTagRenderer("".split(" "), "".split(" "), channelOptions);
StackExchange.using("externalEditor", function()
// Have to fire editor after snippets, if snippets enabled
if (StackExchange.settings.snippets.snippetsEnabled)
StackExchange.using("snippets", function()
createEditor();
);
else
createEditor();
);
function createEditor()
StackExchange.prepareEditor(
heartbeatType: 'answer',
autoActivateHeartbeat: false,
convertImagesToLinks: false,
noModals: true,
showLowRepImageUploadWarning: true,
reputationToPostImages: null,
bindNavPrevention: true,
postfix: "",
imageUploader:
brandingHtml: "Powered by u003ca class="icon-imgur-white" href="https://imgur.com/"u003eu003c/au003e",
contentPolicyHtml: "User contributions licensed under u003ca href="https://creativecommons.org/licenses/by-sa/4.0/"u003ecc by-sa 4.0 with attribution requiredu003c/au003e u003ca href="https://stackoverflow.com/legal/content-policy"u003e(content policy)u003c/au003e",
allowUrls: true
,
onDemand: true,
discardSelector: ".discard-answer"
,immediatelyShowMarkdownHelp:true
);
);
Sign up or log in
StackExchange.ready(function ()
StackExchange.helpers.onClickDraftSave('#login-link');
);
Sign up using Google
Sign up using Facebook
Sign up using Email and Password
Post as a guest
Required, but never shown
StackExchange.ready(
function ()
StackExchange.openid.initPostLogin('.new-post-login', 'https%3a%2f%2for.stackexchange.com%2fquestions%2f2828%2fis-this-constraint-convex%23new-answer', 'question_page');
);
Post as a guest
Required, but never shown
2 Answers
2
active
oldest
votes
2 Answers
2
active
oldest
votes
active
oldest
votes
active
oldest
votes
$begingroup$
Arguments 3 and 4 are incorrect. The Right-Hand Side (RHS) is not convex. Even if it were, setting a nonlinear equality with either side non-affine is non-convex. As the final coup de grace, even if the RHS were convex, an inequality, affine expression $le$ convex RHS, is going the wrong direction to be convex.
I suggest you study sections 2.3 and especially 3.2, both named "Operations that preserve convexity" of Convex Optimization, by Boyd and Vandenberghe which is freely available at the linked website of one of the authors.
$endgroup$
3
$begingroup$
Thank you for the resource! Always appreciate your guidance on the forums.
$endgroup$
– D.Gray
Oct 15 at 16:57
$begingroup$
Boyd also recommends (jokingly?) to work on this problem in two ways, simultaneously: While you try to prove convexity on paper, you run a program that samples two random points, and checks the convexity of the function at their midpoint, potentially providing a counter-example.
$endgroup$
– Robert Schwarz
Oct 16 at 8:41
add a comment
|
$begingroup$
Arguments 3 and 4 are incorrect. The Right-Hand Side (RHS) is not convex. Even if it were, setting a nonlinear equality with either side non-affine is non-convex. As the final coup de grace, even if the RHS were convex, an inequality, affine expression $le$ convex RHS, is going the wrong direction to be convex.
I suggest you study sections 2.3 and especially 3.2, both named "Operations that preserve convexity" of Convex Optimization, by Boyd and Vandenberghe which is freely available at the linked website of one of the authors.
$endgroup$
3
$begingroup$
Thank you for the resource! Always appreciate your guidance on the forums.
$endgroup$
– D.Gray
Oct 15 at 16:57
$begingroup$
Boyd also recommends (jokingly?) to work on this problem in two ways, simultaneously: While you try to prove convexity on paper, you run a program that samples two random points, and checks the convexity of the function at their midpoint, potentially providing a counter-example.
$endgroup$
– Robert Schwarz
Oct 16 at 8:41
add a comment
|
$begingroup$
Arguments 3 and 4 are incorrect. The Right-Hand Side (RHS) is not convex. Even if it were, setting a nonlinear equality with either side non-affine is non-convex. As the final coup de grace, even if the RHS were convex, an inequality, affine expression $le$ convex RHS, is going the wrong direction to be convex.
I suggest you study sections 2.3 and especially 3.2, both named "Operations that preserve convexity" of Convex Optimization, by Boyd and Vandenberghe which is freely available at the linked website of one of the authors.
$endgroup$
Arguments 3 and 4 are incorrect. The Right-Hand Side (RHS) is not convex. Even if it were, setting a nonlinear equality with either side non-affine is non-convex. As the final coup de grace, even if the RHS were convex, an inequality, affine expression $le$ convex RHS, is going the wrong direction to be convex.
I suggest you study sections 2.3 and especially 3.2, both named "Operations that preserve convexity" of Convex Optimization, by Boyd and Vandenberghe which is freely available at the linked website of one of the authors.
edited Oct 15 at 17:07
answered Oct 15 at 16:01


Mark L. StoneMark L. Stone
4,7151 gold badge10 silver badges35 bronze badges
4,7151 gold badge10 silver badges35 bronze badges
3
$begingroup$
Thank you for the resource! Always appreciate your guidance on the forums.
$endgroup$
– D.Gray
Oct 15 at 16:57
$begingroup$
Boyd also recommends (jokingly?) to work on this problem in two ways, simultaneously: While you try to prove convexity on paper, you run a program that samples two random points, and checks the convexity of the function at their midpoint, potentially providing a counter-example.
$endgroup$
– Robert Schwarz
Oct 16 at 8:41
add a comment
|
3
$begingroup$
Thank you for the resource! Always appreciate your guidance on the forums.
$endgroup$
– D.Gray
Oct 15 at 16:57
$begingroup$
Boyd also recommends (jokingly?) to work on this problem in two ways, simultaneously: While you try to prove convexity on paper, you run a program that samples two random points, and checks the convexity of the function at their midpoint, potentially providing a counter-example.
$endgroup$
– Robert Schwarz
Oct 16 at 8:41
3
3
$begingroup$
Thank you for the resource! Always appreciate your guidance on the forums.
$endgroup$
– D.Gray
Oct 15 at 16:57
$begingroup$
Thank you for the resource! Always appreciate your guidance on the forums.
$endgroup$
– D.Gray
Oct 15 at 16:57
$begingroup$
Boyd also recommends (jokingly?) to work on this problem in two ways, simultaneously: While you try to prove convexity on paper, you run a program that samples two random points, and checks the convexity of the function at their midpoint, potentially providing a counter-example.
$endgroup$
– Robert Schwarz
Oct 16 at 8:41
$begingroup$
Boyd also recommends (jokingly?) to work on this problem in two ways, simultaneously: While you try to prove convexity on paper, you run a program that samples two random points, and checks the convexity of the function at their midpoint, potentially providing a counter-example.
$endgroup$
– Robert Schwarz
Oct 16 at 8:41
add a comment
|
$begingroup$
Counterexamples to your arguments:
Argument 1:
Only affine equality constraints are convex, $x = y^2$ is not convex.
Argument 3:
Take $f(x) = x^4$ and $g(x) = x$. Both are convex, but the ratio $h(x) = fracx^4x $ is not.
Argument 4:
Let $f(x) = x$, and $y in mathbbR$. $f$ is convex, but $g(x, y) = yf(x) = xy$ is not.
$endgroup$
add a comment
|
$begingroup$
Counterexamples to your arguments:
Argument 1:
Only affine equality constraints are convex, $x = y^2$ is not convex.
Argument 3:
Take $f(x) = x^4$ and $g(x) = x$. Both are convex, but the ratio $h(x) = fracx^4x $ is not.
Argument 4:
Let $f(x) = x$, and $y in mathbbR$. $f$ is convex, but $g(x, y) = yf(x) = xy$ is not.
$endgroup$
add a comment
|
$begingroup$
Counterexamples to your arguments:
Argument 1:
Only affine equality constraints are convex, $x = y^2$ is not convex.
Argument 3:
Take $f(x) = x^4$ and $g(x) = x$. Both are convex, but the ratio $h(x) = fracx^4x $ is not.
Argument 4:
Let $f(x) = x$, and $y in mathbbR$. $f$ is convex, but $g(x, y) = yf(x) = xy$ is not.
$endgroup$
Counterexamples to your arguments:
Argument 1:
Only affine equality constraints are convex, $x = y^2$ is not convex.
Argument 3:
Take $f(x) = x^4$ and $g(x) = x$. Both are convex, but the ratio $h(x) = fracx^4x $ is not.
Argument 4:
Let $f(x) = x$, and $y in mathbbR$. $f$ is convex, but $g(x, y) = yf(x) = xy$ is not.
answered Oct 15 at 18:55


Michael FeldmeierMichael Feldmeier
2,5096 silver badges37 bronze badges
2,5096 silver badges37 bronze badges
add a comment
|
add a comment
|
Thanks for contributing an answer to Operations Research Stack Exchange!
- Please be sure to answer the question. Provide details and share your research!
But avoid …
- Asking for help, clarification, or responding to other answers.
- Making statements based on opinion; back them up with references or personal experience.
Use MathJax to format equations. MathJax reference.
To learn more, see our tips on writing great answers.
Sign up or log in
StackExchange.ready(function ()
StackExchange.helpers.onClickDraftSave('#login-link');
);
Sign up using Google
Sign up using Facebook
Sign up using Email and Password
Post as a guest
Required, but never shown
StackExchange.ready(
function ()
StackExchange.openid.initPostLogin('.new-post-login', 'https%3a%2f%2for.stackexchange.com%2fquestions%2f2828%2fis-this-constraint-convex%23new-answer', 'question_page');
);
Post as a guest
Required, but never shown
Sign up or log in
StackExchange.ready(function ()
StackExchange.helpers.onClickDraftSave('#login-link');
);
Sign up using Google
Sign up using Facebook
Sign up using Email and Password
Post as a guest
Required, but never shown
Sign up or log in
StackExchange.ready(function ()
StackExchange.helpers.onClickDraftSave('#login-link');
);
Sign up using Google
Sign up using Facebook
Sign up using Email and Password
Post as a guest
Required, but never shown
Sign up or log in
StackExchange.ready(function ()
StackExchange.helpers.onClickDraftSave('#login-link');
);
Sign up using Google
Sign up using Facebook
Sign up using Email and Password
Sign up using Google
Sign up using Facebook
Sign up using Email and Password
Post as a guest
Required, but never shown
Required, but never shown
Required, but never shown
Required, but never shown
Required, but never shown
Required, but never shown
Required, but never shown
Required, but never shown
Required, but never shown
i C,Po,N,zdpYGbttTMZtuS,CA4iJiizHEA,kdiDA
$begingroup$
Even though your argument is not correct, there is still a possibility to obtain a convex formulation. You could substitute all your $X_t$ variables using the equality constraints that you showed us. If you are (really) lucky, it might be that your problem is convex after the substitutions.
$endgroup$
– Kevin Dalmeijer
Oct 16 at 3:20